Insights
if ( ! empty( $excerpt ) ) :?>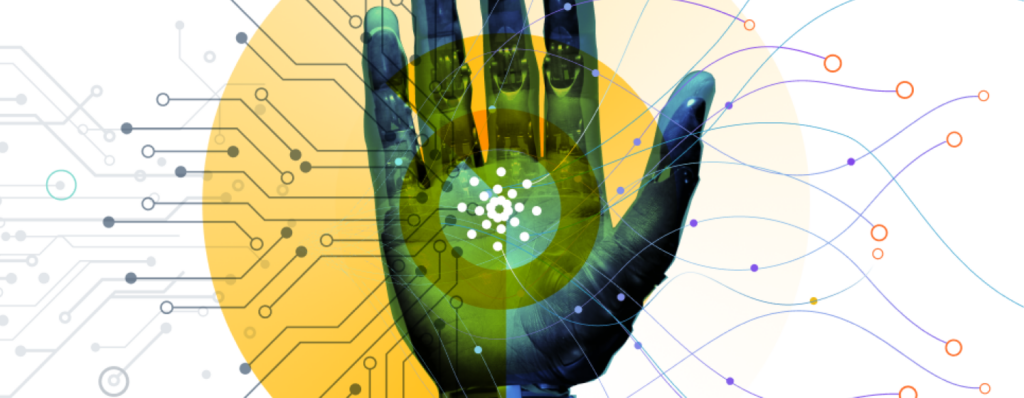
Featured Insights
What’s happening in the global communication space? Is your business ready to scale with that latest technologies? Catch up on industry news and see what experts have to say.
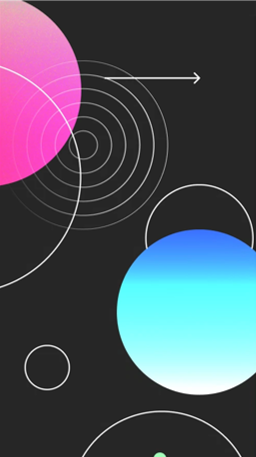
AI in Action: Combining People, Processes, and Technology
Introduction AI in Action Combining People, Processes, and Technology We…
Related Content
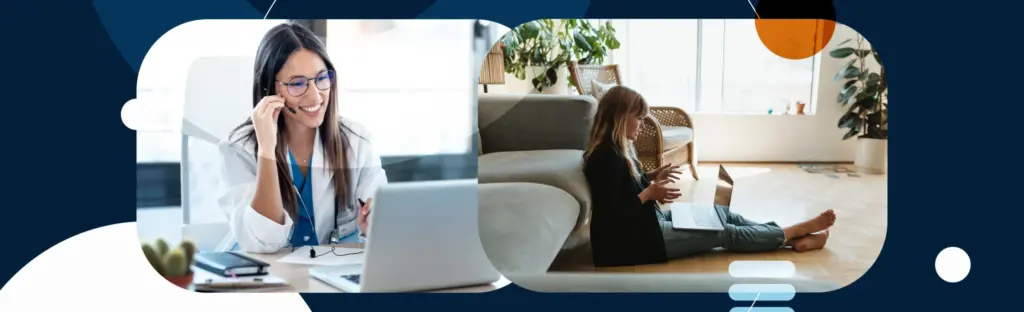
Revolutionizing Patient Engagement: The Transformative Power of AI in Healthcare
The field of healthcare is rapidly evolving, and artificial intelligence…
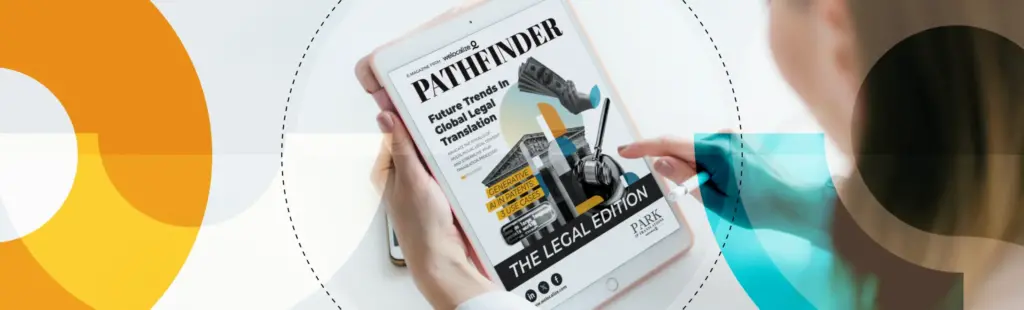
PATHFINDER – THE LEGAL EDITION
Welcome to the legal edition of PATHFINDER, an e-magazine exploring…
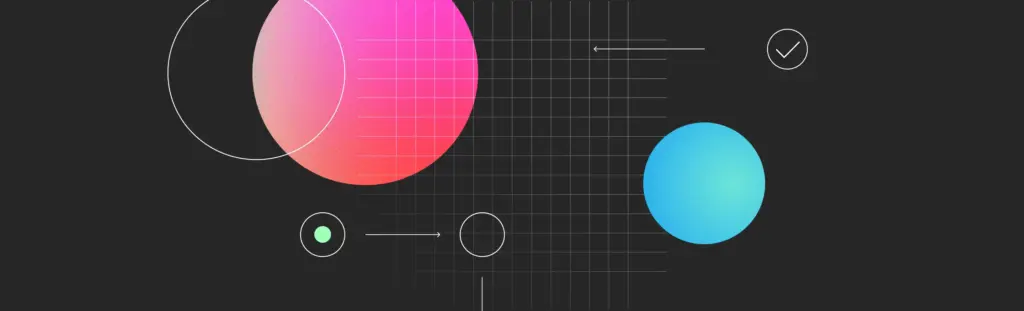
AI in Action: Combining People, Processes, and Technology
We live in an AI-driven content transformation era that is…
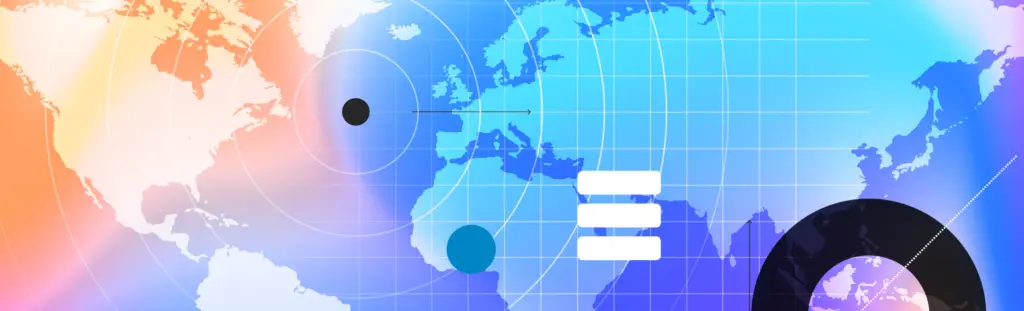
CASE STUDY: Revolutionizing Marketing Localization for Global Tech Giant
This case study explores how a leading global technology company…
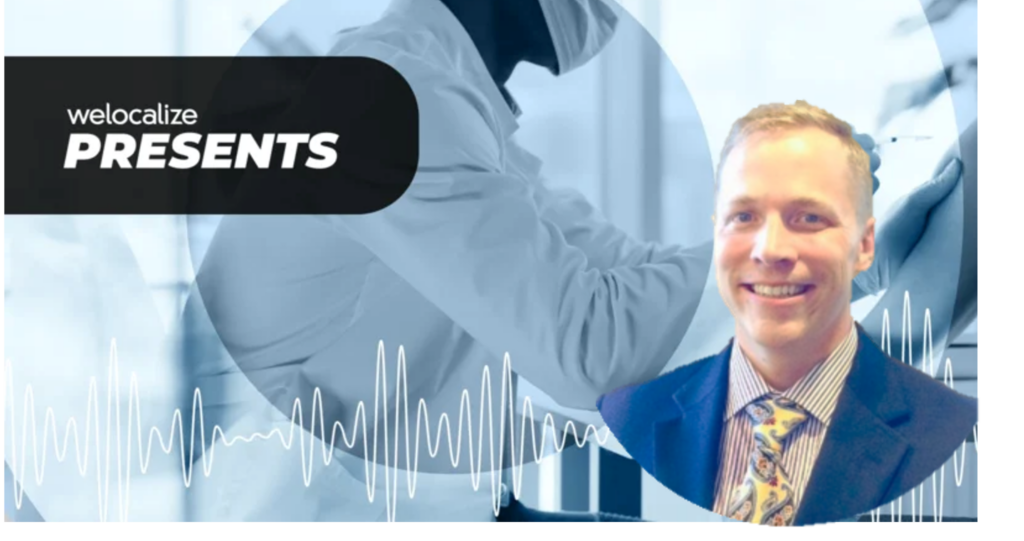
Inside Rare Disease Clinical Trials: Podcast Episode 10 with Joe Tyler
In the tenth episode of Welocalize Presents, host Louise Law chats with Joe…
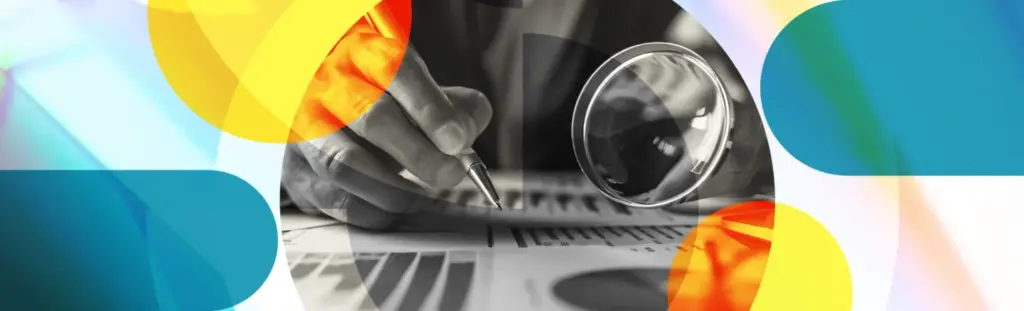
From Language Barriers to Legal Compliance: How AI is Changing the Game
In an era marked by rapid globalization and digital transformation,…
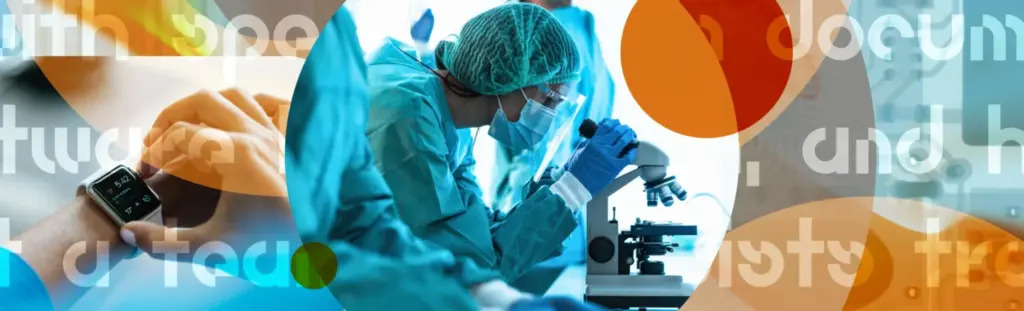
CASE STUDY: Global Growth for Leading Company in 34+ Languages
This case study explores how a leading medical technology company…
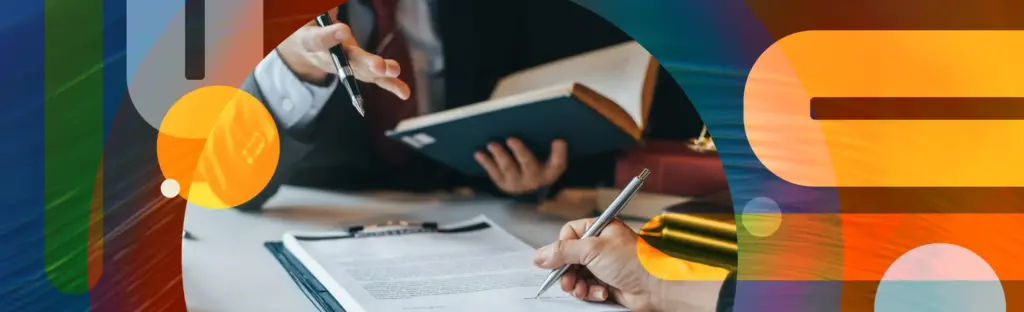
Multilingual Litigation in the Age of AI
In today’s global economy, businesses are doing more than just…
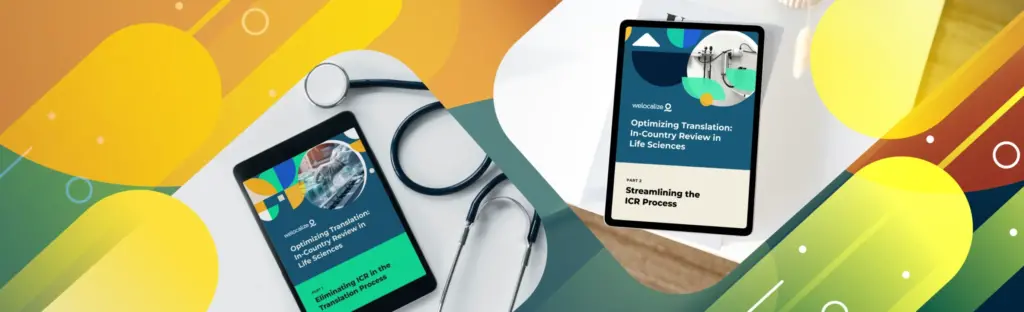
Transforming Translation in Life Sciences: Rethinking ICR
In the dynamic field of life sciences, the accuracy and…
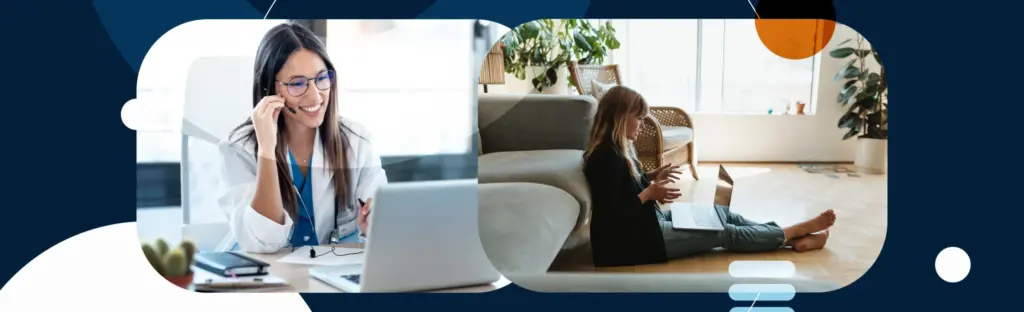
Revolutionizing Patient Engagement: The Transformative Power of AI in Healthcare
The field of healthcare is rapidly evolving, and artificial intelligence…
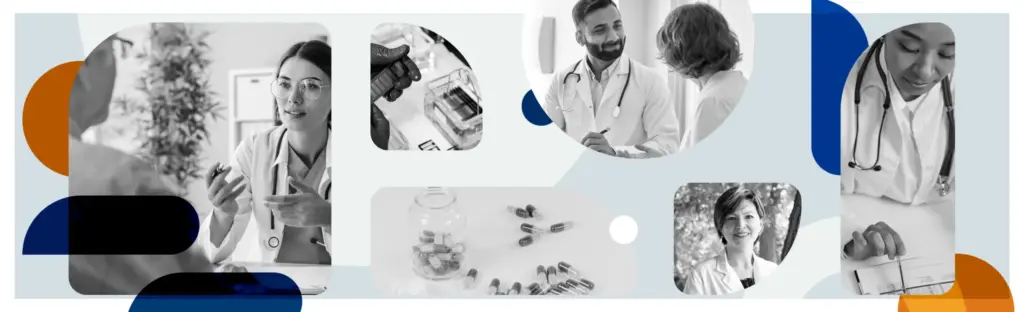
Enhancing Diversity in Clinical Trials: The Transformative Role of AI
In today’s fast-paced medical landscape, the call for diversity in…
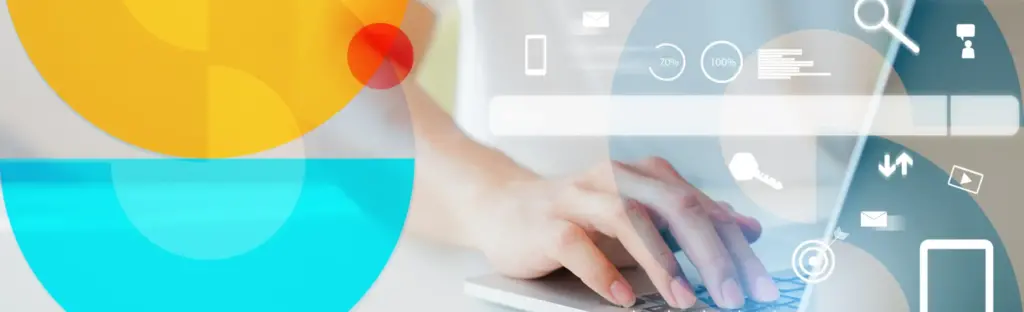
Global Tech Brand Serves Relevant Content to International Customers
Global tech brand engages Welocalize to enhance its content relevance…