
Imagine waking up to a world where your morning alarm intuitively adjusts to your sleep cycle, your coffee maker knows just when to start brewing, and your digital assistant schedules your day flawlessly, all thanks to artificial intelligence (AI).
At the core of any AI’s learning process is data — sometimes vast amounts of it, other times smaller curated sets.
AI is only as good as the data it’s trained on. That’s where data annotators and evaluators step in.
While advancements in synthetic data and auto-training are impressive, they cannot fully replicate human capabilities.
The narrative surrounding the human workforce behind AI often focuses on the challenges and pitfalls of so-called ghost work.
The narrative surrounding the human workforce behind AI often focuses on the challenges and pitfalls of so-called ghost work. Fortunately, the language services industry is redefining the data annotation profession, proving it can be a positive and rewarding career.
When AI development considers the well-being of the human workforce behind it, the industry fosters trust and transparency throughout the process. Prioritizing fair treatment and compensation ensures the integrity and reliability of the data used to train AI models, leading to more accurate and trustworthy results.
The meticulous work of human labeling isn’t simply a necessary step; it’s the cornerstone of accurate and effective AI. Investing in a responsible approach to data annotation isn’t just the right thing to do; it’s a strategic advantage.
Welo Data
Welocalize employs a global workforce and network of experts fluent in various languages. This enables us to accurately label diverse data sets, which is crucial for training AI models that function across different cultures.
Blending machine automation, human intelligence, and an understanding of 250+ languages, Welocalize will help you unlock the value of multilingual training data to power chatbots, digital assistants, search engines, voice interfaces, and much more.
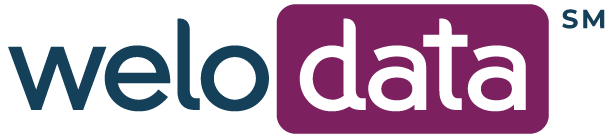
