
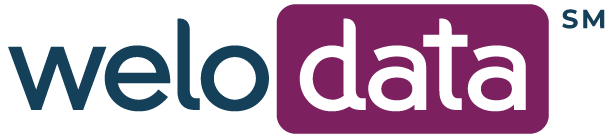
Behind the Code
The Human Force Powering
AI Innovation
Discover how the human touch transforms AI innovation, ensuring accuracy and effectiveness through meticulous data labeling.
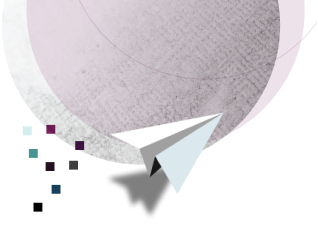
Imagine waking up to a world where your morning alarm intuitively adjusts to your sleep cycle; your coffee maker knows when to start brewing, and your digital assistant schedules your day flawlessly, all thanks to AI.
At the core of any AI’s learning process is data — sometimes vast amounts of it, other times smaller curated sets.
AI is only as good as the data it’s trained on. That’s where data annotators and evaluators step in. Their meticulous work ensures the data fed to AI is accurate, nuanced, and culturally sensitive.
While advancements in synthetic data and auto-training are impressive, they cannot fully replicate human capabilities.
The narrative surrounding the human workforce behind AI often focuses on the challenges and pitfalls of so-called ghost work.
Introduction
Imagine waking up to a world where your morning alarm intuitively adjusts to your sleep cycle; your coffee maker knows just when to start brewing, and your digital assistant schedules your day flawlessly, all thanks to artificial intelligence (AI).
Behind these omnipresent technologies lies a critical, often overlooked process: data labeling. This meticulous task, performed by countless unseen human hands, involves tagging raw data—like images, audio clips, and text—with informative labels, turning it into a signal AI can understand and learn from.
The human touch in data annotation isn’t just a step in the process; it’s the very foundation upon which accurate and effective AI is built. This meticulous process tags raw data with metadata, allowing machine learning (ML) models to understand and learn from it. Examples are labeling a picture “car” or an audio clip “customer support call.”
These models empower advanced applications such as chatbots, autonomous vehicles, and speech recognition systems like your in-home Assistant speakers. Recent research highlights the evolving landscape where large language models (LLMs) can substitute human labelers for straightforward classification tasks.
However, the importance of consent-based, human-labeled data remains paramount, especially in complex scenarios where nuanced understanding and ethical considerations are critical.
