
BEHIND THE CODE | Chapter 2
The Vital Role of Data
Annotation and Labeling
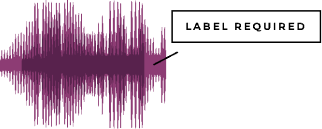
Imagine waking up to a world where your morning alarm intuitively adjusts to your sleep cycle; your coffee maker knows just when to start brewing, and your digital assistant schedules your day flawlessly, all thanks to AI.
At the core of any AI’s learning process is data — sometimes vast amounts of it, other times smaller curated sets.
AI is only as good as the data it’s trained on. That’s where data annotators and evaluators step in.
While advancements in synthetic data and auto-training are impressive, they cannot fully replicate human capabilities.
The narrative surrounding the human workforce behind AI often focuses on the challenges and pitfalls of so-called ghost work.
At the core of any AI’s learning process is data — sometimes vast amounts of it, other times smaller curated sets. For AI to effectively understand and process this data, it often benefits from being annotated or labeled, which involves tagging images, text, audio, and video with metadata that AI can comprehend.
However, AI can also learn through unsupervised methods without explicit annotations, enhancing its ability to recognize patterns and make decisions mirroring human cognition.
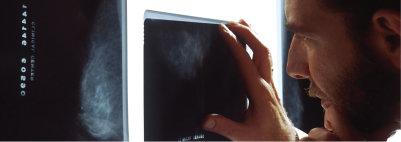
Data annotation isn’t just a mechanical checkbox task. It requires a skilled workforce with a keen eye and the ability to think beyond the label. Here’s why:
- Accuracy is paramount: Inaccurate data labeling can disrupt the entire operational framework of AI systems, emphasizing the need for precision in data annotation.
- Cultural nuances matter: Understanding regional slang or humor helps the AI model navigate the complexities of human communication.
- Domain expertise is key: A doctor labeling medical scans needs a different skill set than someone labeling car parts for self-driving vehicles.
