
Imagine waking up to a world where your morning alarm intuitively adjusts to your sleep cycle; your coffee maker knows just when to start brewing, and your digital assistant schedules your day flawlessly, all thanks to AI.
At the core of any AI’s learning process is data — sometimes vast amounts of it, other times smaller curated sets.
AI is only as good as the data it’s trained on. That’s where data annotators and evaluators step in.
While advancements in synthetic data and auto-training are impressive, they cannot fully replicate human capabilities.
The narrative surrounding the human workforce behind AI often focuses on the challenges and pitfalls of so-called ghost work.
While advancements in synthetic data and auto-training are impressive, they cannot fully replicate human capabilities. Algorithms struggle to grasp the underlying intent and meaning behind data points, often leading to misinterpretations. Human annotators have distinct advantages:
Superior Nuance and Accuracy
Humans possess an innate ability to understand context, cultural subtleties, and the complexities of language. This allows them to accurately label ambiguous data points that synthetic data or auto-training might misinterpret.
Domain Expertise
For tasks requiring specialized knowledge (e.g., medical diagnosis, legal document analysis), human experts can provide a level of precision that algorithmic labeling often struggles to achieve.
Addressing Bias
Synthetic data can perpetuate existing biases present in the data it’s derived from. Human labelers can identify and mitigate these biases, ensuring the fairness and generalizability of the trained model.
Real-World Applicability
The ability to label real-world data with its inherent messiness and inconsistencies better prepares AI models for functioning effectively in unsensitized environments.
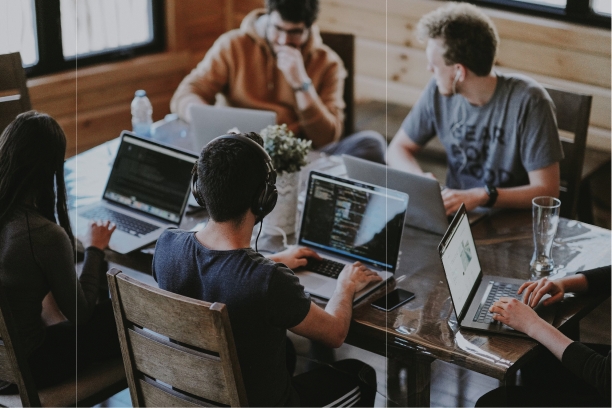
.
Accountability
Humans are accountable for the quality of their annotations and can undergo training to enhance their performance. In contrast, data annotation tools, operating without human oversight, cannot be held liable for biases, errors, or misrepresentations present in the labeled data.
Quality Assurance
Data labeling relies heavily on quality assurance to ensure the success of the machine learning model. This involves achieving a ground-truth level of accuracy, uniqueness, independence, and information. Human involvement is crucial in this process, and humans can provide more accurate and meaningful insights for quality control than machines.
Flexibility
Given the dynamic nature of both internal and external variables, it may be necessary to adjust labeling guidelines or project requirements. Human data labeling offers the flexibility sometimes needed in the labeling process.
So, while synthetic data and auto-training are powerful tools that make the process scalable, human annotation remains crucial for ensuring the accuracy, fairness, and real-world applicability of ML models.
