Training Data: Its Role in Multilingual AI Performance
The global conversational AI market is projected to reach $41.4 billion by 2030, growing by 23.6% compounded yearly from 2022 to 2030. Chatbots and interactive virtual assistants have come a long way from the early days of rules-based systems with canned responses. Artificial intelligence (AI) has enabled chatbots and voice assistants to understand and converse…
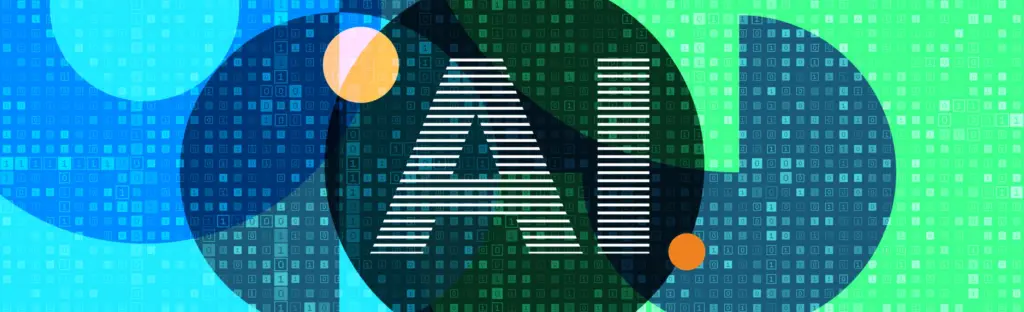
The global conversational AI market is projected to reach $41.4 billion by 2030, growing by 23.6% compounded yearly from 2022 to 2030. Chatbots and interactive virtual assistants have come a long way from the early days of rules-based systems with canned responses.
Artificial intelligence (AI) has enabled chatbots and voice assistants to understand and converse in natural language, even in multiple languages. While building AI models is crucial in the development of conversational AI, what drives its uncanny accuracy and human-like responses is AI training data.
What Is AI Training Data?
AI training data is the foundation of AI development. AI chatbots and other applications do not exist in a vacuum. AI algorithms need vast amounts of data to learn and recognize patterns, make decisions, and solve problems. Machine learning is a subfield of AI that enables machines like chatbots to learn from data and past experiences on their own; in other words, to learn like humans.
This data includes text, images, audio, video, sensor data, and language translations for multilingual conversational AI. The quality and quantity of that data play a critical role in determining the accuracy and effectiveness of the AI model. The more relevant and diverse data sources are fed to the AI algorithm, the greater its ability to learn and perform designated tasks with increasing accuracy and efficiency.
AI training data is used for numerous AI use cases in the following ways:
- Learning and recognition: Training data provides AI algorithms with the information they need to learn and recognize patterns.
- Problem-solving: AI algorithms are designed to solve problems by analyzing and making predictions based on data.
- Natural language processing: Training data is crucial in natural language processing, where AI models are designed to understand human language, including its nuances, and respond appropriately.
- Personalization: Personalized AI models that cater to individual needs, such as an AI customer service chatbot, must be trained on vast customer data to understand their needs and provide personalized responses.
Benefits of AI Training Data for Global Brands
Conversational AI requires well-designed AI models and accurate training data to be effective. Global brands can benefit in several ways. These benefits include:
- Better customer experience: Well-trained chatbots can understand user intent and provide more accurate and relevant responses, which leads to higher customer satisfaction.
- Enhanced brand image: Customers are more likely to trust a brand that provides high-quality customer support, including from AI chatbots, and is responsive to their needs.
- Reduction in costs: Accurate conversational AI systems can provide automated customer support, passing on more complex queries to human support agents. By needing fewer human agents, global brands can lower their operational costs.
- Increased efficiency: AI chatbots are available 24/7 and can handle large volumes of customer queries at the same time, reducing wait times and improving response times.
- Multilingual support: Global brands can benefit from good data by training conversational AI systems to provide customer support in different languages.
Machine Translation and Training Data for Multilingual Output
Machine translation (MT), a subset of AI, uses machine learning algorithms to translate text or speech from one language to another automatically. Machine translation systems rely on large volumes of high-quality training data to produce high-quality translations.
The training data for MT typically consists of parallel corpora, which are pairs of sentences in the source language and their corresponding translations in the target language. The more diverse and high-quality the parallel corpora are, the better the MT system will be.
Once the training data is created, it’s used to train MT models using supervised learning techniques. These models learn how to translate text from the source language to the target language by analyzing patterns and relationships within the training data.
This is a continuous process that requires fine-tuning the MT models, adding new data to the training set, or adjusting the translation rules better to capture the nuances of the source and target languages.
Bad Input = Bad Output: Challenges in Creating AI Training Data
Conversational AI performance is only as good as the data it is fed. Bad input can result in bad output in AI data training because the quality and relevance of the training data directly impact the accuracy and effectiveness of the AI model. If the training data is biased, incomplete, or irrelevant to the designated task, the model will not learn the correct patterns or make accurate predictions.
The problems with bad data are exacerbated by having too much data. While a large amount of data is beneficial in training AI models, it can also present several challenges that must be overcome.
- Data storage and processing: A massive volume of data requires significantly more storage capacity and computational power to process the data efficiently. Specialized hardware and software infrastructure can be complex and costly.
- Data quality and relevance: It can be more challenging to ensure the quality and relevance of the data when there is too much of it. It will involve time-consuming cleaning and pre-processing of the data, which requires resources, specialized tools, and techniques.
- Bias and fairness: There is always potential for bias and discrimination in the training data. Biased data can result in AI models that discriminate against certain groups of people or produce inaccurate results.
- Interoperability: The data used for AI training often comes from various sources and is stored in different formats, making it difficult to integrate and combine the data effectively. This can hinder interoperability between different AI systems.
- Overfitting: Bad input can lead to overfitting, where the AI model learns the training data too well and cannot generalize to new data. This can happen when the training data is too specific or limited, and the model has not been exposed to a diverse set of examples.
- Ethics and privacy: As the amount of data collected and used for AI training continues to grow, there is an increased risk of privacy breaches and ethical concerns. Sensitive data must be protected, and privacy regulations must be followed to ensure the use of data is ethical and legal.
How to Create Training Data for Conversational AI
Creating training data for conversational AI involves several steps, including defining the use case, designing the conversational flow, and labeling the data. Here’s an overview of the process:
- Define the use case: What is the use case for the conversational AI system? Identify the problem the system will solve and the target audience. Have a clear understanding of the use case before creating the training data.
- Design the conversational flow: This involves identifying the different stages of the conversation and the types of questions and responses needed to move the conversation forward. The conversational flow should be designed to meet the needs of the target audience and to achieve the desired outcome.
- Create sample conversations: Using the conversational flow as a guide, create sample conversations representing the types of interactions that users are likely to have with the conversational AI system. These conversations should be as natural and varied as possible, including different question types, tones, and styles.
- Label the data: Labeling AI training data involves annotating data with meaningful and relevant labels that help a machine learning model learn from the data. For example, if a user asks for the weather forecast, the intent would be “get weather forecast,” and the relevant information would be the date, time, and location. Minimize the introduction of bias into the labeled data by using diverse annotators.
- Use natural language processing: Conversational AI systems rely on natural language processing (NLP) to understand and respond to user messages. NLP involves analyzing the user’s message to identify the intent and extract the relevant information. Use NLP tools and techniques to label the data.
- Validate the data: After labeling it, validate the data and annotations to ensure they are accurate and complete. This includes checking for errors, inconsistencies, and missing information. Involve a diverse set of users to ensure it meets the target audience’s needs.
- Continuously iterate: Creating training data for conversational AI is an ongoing process. As the system is deployed and used, continue to evaluate, and refine the data to ensure it meets the needs of the users and the use case.
Data labeling is a critical step in AI development, but it also raises ethical and legal concerns that must be addressed. For example, data labeling may involve sensitive data, and it’s essential to ensure the privacy and confidentiality of individuals are protected. If it involves personal data, you must obtain informed consent from users and be transparent in explaining how their data will be used.
You should also exert much effort to prevent bias in data labeling so AI models don’t perpetuate or amplify existing biases in the data. Be consistent and accurate by developing clear guidelines and standards for the labeling process.
After creating the training data, test the model using the labeled data to evaluate its accuracy and generalization to new, unseen data. If the model isn’t performing well, you may need to revisit the annotation process and make further improvements.