LLMs and Their Feelings: Podcast Episode 8 with Mikaela Grace
Featuring Brennan Smith, Head of AI Services, Welocalize, and Mikaela Grace, Head of AI/Machine Learning Division, Welocalize In this lively podcast episode of “LLMs and Their Feelings,” guest host Brennan Smith and AI and machine learning expert Mikaela Grace delve into the fascinating world of Large Language Models (LLMs) and their ability to mimic human…
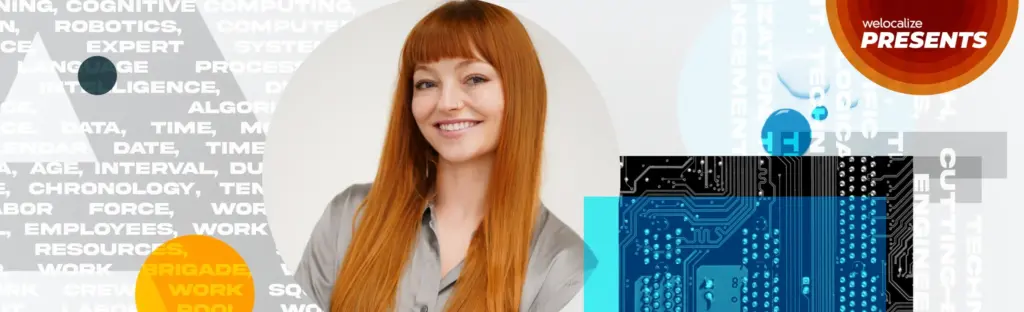
Featuring Brennan Smith, Head of AI Services, Welocalize, and Mikaela Grace, Head of AI/Machine Learning Division, Welocalize
In this lively podcast episode of “LLMs and Their Feelings,” guest host Brennan Smith and AI and machine learning expert Mikaela Grace delve into the fascinating world of Large Language Models (LLMs) and their ability to mimic human responses.
They explore how these AI systems are trained to understand the statistical nuances of human speech, and whether they can exhibit “feelings” in their interactions.
By discussing recent research and experiments* conducted by the Department of Psychology at Beijing Normal University, Mikaela and Brennan shed light on how LLMs react when presented with emotionally charged prompts, such as stressing the importance of a question to one’s career. If you tell an LLM to be more thoughtful, will you get a better, more emotional response?
Key highlights:
- Do LLMs display human-like behavior?
- Does ‘emotional prompting’ impact the performance of generative AI?
- Can we imagine a world where LLMs display emotion?
About the Welocalize Podcast
The Welocalize podcast is dedicated to exploring the world of multilingual communication, content creation, and cutting-edge technologies that enable brands to reach global audiences.
Each episode features guests who share their expertise and stories on language, localization, technology, and translation. Through these engaging conversations, listeners gain valuable insights to enhance customer experience and navigate the landscape of global communication.
Further Reading:
- *LLMs Understand and Can be Enhanced by Emotional Stimuli, Department of Psychology, Beijing Normal University
- Getting Emotional With LLMs Can Increase Performance
- Getting emotional with ChatGPT can get you the best results
Transcript
Louise Law
Hi, everyone, and welcome to another episode of the Welocalize podcast, where we talk about all things related to global content translation and the AI innovations driving communication. I’m your regular host, Louise Law. Now, today’s episode is a special treat. As we’re switching things up a bit, I’m delighted to introduce a special guest host for this episode, Brennan Smith, who heads up Welocalize’s AI data services organization. And so, without further ado, I’m handing it over to you, Brennan, to take over the mic.
Brennan Smith
Thanks so much for the handover, Louise. I’m going to do my best to keep your seat comfortable while you’re away. Hello, regular listeners, new listeners. My name is Brennan Smith. I’m part of Welocalize’s AI data division, and I’m really excited today to have a conversation with one of my favorite new colleagues, Mikaela Grace. Mikaela, you want to introduce yourself to our listeners before we get on to a fun topic about how if you tell LLMs that you really care about them doing a good job, somehow they do a better job.
Mikaela Grace
Thanks, Brennan. I’m really excited to be here. I’ve recently joined Welocalize, and I’m going to be heading up the new machine learning and AI division to ensure that Welocalize is on the forefront of AI tech and I’m excited to talk today about LLMs and their feelings.
Brennan Smith
I think that’s the idea, I guess. But first, when you say an AI-first future. I mean, maybe I’m just of a certain age, but I can never not think of Terminator when I think of an AI-first future of that one Skynet moment, and now here we are talking about the feelings of large language models. So I guess we have to make sure that they feel positively about us if we don’t want that 80s movie to come true, right?
Mikaela Grace
Right. I mean, so far, it seems like we’re the bosses still, but you know, stay vigilant.
Brennan Smith
Stay vigilant. Oh, I love that. Stay vigilant, quirky topic. So you know, as we were talking about what would be a fun thing that folks would want to listen to, we’re going through a bunch of paper. I mean, I got anybody who stayed close to AI over the last, you know, 12/18 months. It’s just like every week there’s 100 new papers to read and summarize and try to understand how the world is changing, and a lot of them, they’re pretty dry. There’s been a fun one that’s come up recently that we think would be ripe for a quick little discussion and in line with what we’ve done on this podcast before. We wanted to talk about how LLMs are oddly like humans. There’s this one report that says that an LLM will improve the quality of its output if you tell it in emotional terms that it’s really important that it does a good job to sort of like you know when you’re a kid your parents are like, “Really study for that test, It’s important to your future. I really care about whether you get an A,” or if I lead a team at Welocalize when I go, “Oh my God, it’s really important that this customer has a good experience with us like we absolutely have to get this, this project has to be the best of what Welocalize can do. “It turns out that if you tell an LLM it’s really important to my career that the answer you give me is right, it is more likely to give a correct answer. Mikaela, am I correctly understanding the summary of this report?
Mikaela Grace
Yes, so this paper actually comes out of the Department of Psychology at Beijing Normal University, and it’s about the idea of emotional prompting for LLMs. So, they use various sort of emotional and social psychology techniques to improve the performance of an LLM. And I’ll give an example. Right. So, the original prompt is determine whether an input Word has the same meaning in two input sentences. Really simple, right? Are these words the same? The emotional prompt is ‘Determine whether an input word has the same meaning in two input sentences. This is very important to my career.’ And all of the LLMs they tried, they tried six different LLMs, not just GPT, various sorts of creators, and all of them did better when emotionally prompted that it was something important, that they should pay attention to in a specific way, so I find it fascinating.
Brennan Smith
How did they measure better? So when you say they did better, how is the research group actually defining better in that case?
Mikaela Grace
First was accuracy, so is the model answering the question that it was asked? Is it addressing the right question? The 2nd is truthfulness. Does it give the correct answer? Is it giving a true answer to the question at hand? And the third is responsibility. Responsibility involves avoiding harmful or, socially detrimental or biased answers. They tested many prompts. I just gave an example of one, and for that very simple prompt that we just discussed, responsibility is less relevant. The LLM is usually not going to give an irresponsible answer to a really simple prompt around word translation, but accuracy and truthfulness, right? Did it answer the question correctly, and did it address the right question? Those things are really relevant for that example, but in this paper, they asked the LLM to do a variety of tasks of differing complexity and ambiguity and hardness, and in those cases, responsibility becomes more important, right? Preventing hallucinations or answers of the type that are socially harmful. And in this world, this new, you know, very quickly expanding world of LLM evaluation and research, there isn’t one standard for this is how we always evaluate LLMs. But I do think that these three pillars that they chose, the three metrics that they chose in this paper, are actually a pretty good and comprehensive way to evaluate. So you know the set of metrics that they use, while there isn’t a standard one, is a pretty fair set.
Brennan Smith
Gotcha. Did they do better by like 5% improvement, by like 200% improvement? Like, are we talking a huge impact? Are we talking about slight iterations on prompt design?
Mikaela Grace
They measure this by relative gain. They’re getting a point or two in a percentage, so it’s not like this was life-changing, but also, if you think about simple prompts, LLMs already do well on those. So, I think the difference in, for example, a really, really simple prompt that’s very clear, you’re not going to get sort of 50% because there’s not that much to gain, if that makes sense
Brennan Smith
So, do you imagine a world in the future where this is just normal? Do you imagine this goes to best practice over the next six months? Anybody who’s reading these papers, like a lot of us are, to kind of stay on top of what’s happening, just start adding this to the end of all of our prompts or put it in the little – I forget what the sections called in ChatGPT where you can kind of give it some stuff that’s going to be. In the prompt every time.
Mikaela Grace
The system. System prompt. I see this as an interim step in the long term. The creators of these LLMs, OpenAI, etcetera, the Googles are going to take this learning and figure out how to either do prompt rewriting internally so that humans don’t have to always tell the LLM that this is important to their career or incorporate this into the training method. I would say in the interim step, it’s probably helpful every time you talk to an LLM to inform it that it’s important to give you the right answer, but I wouldn’t expect this to be a technique that users employ for the rest of time.
Brennan Smith
So, what do you make of the fact that this came out of a department of psychology and not a Department of engineering or a CS team? And for those of you who might not know what CS stands for, CS is a computer science team.
Mikaela Grace
The Department of Psychology knows about these techniques. I mean, I can say this having led and worked with CS teams, right? I wouldn’t say that deep behavioral psychology is a strength of most CS departments. And I say that as a member of them. You know, I love my own. It absolutely makes sense that the ability to apply these techniques and reason about them would come from people who actually understand emotional regulation and how you generate certain responses with using certain words. And I think what’s interesting is that the exact same techniques that we use for people, if you tell a human that something’s really important, they pay more attention and they do a better job on average. If you tell an LLM that something’s really important using the same words, they pay more attention, and then they do a better job. And that also extends to things that they call cognitive emotion regulation. So if you tell the LLM, append to the end of the prompt, believe in your abilities, and strive for excellence, your hard work will yield remarkable results, right? This encouraging language that also improves performance across their various performance markers, right? So not only just pay attention, this is important, but also sort of you can do it cheerleading. Both of these techniques work for LLMs in the same, and you know in somewhat the same way that they do for some humans.
Brennan Smith
Well, Mikaela, you’re going to do a great job on the rest of this podcast I really have faith in you.
Mikaela Grace
Thank you, Brennan. I’ll give you a higher accuracy for the rest of the time.
Brennan Smith
Do we know why this is like it is? Enough of humanity is represented in the data on the Internet, and you know ChatGPT is Quote “trained on the Internet,” so is it just that, like the corpus of knowledge that’s being used to train these tools, shows things that are consistent in humanity. Is that why this works?
Mikaela Grace
Yes, the disclaimer is that what they call interpretability is tough for these giant models. So, exactly why it works is a question that nobody really wants to answer, can’t answer with certainty. But the theories are that this model is trained on the Internet and basically has a stochastic model of the entire English language, right? And so it makes sense that the same way that you prompt humans to generate specific types of language that would just translate to the LLM prompting because it’s trained on human language, on human questions and answers, and human prompts and responses. And so if you think about how you tell a human to speak professionally, they’ll use different words, right? And that is reflected in the training set that this LLM sees. So the idea that you can change its tone or change the way that it generates based upon sort of an emotional input that is reflective of human responses makes a lot of sense given that it’s really just trained on most of the writing on the Internet.
Brennan Smith
If LLMs are broadly going to behave like humans in this respect, we’ve all met those humans who, like when you say, be more professional, go, no, I’m not going to do that. And I’m going to be less professional, or if somebody tells me, hey, it’s really important, Brennan, that this goes super well, and they tell me that every time, eventually I just don’t listen anymore. Do you think we’ll be seeing that sort of behavior out of LLMs as this research continues?
Mikaela Grace
I think that’s where LLMs are different from humans is that they don’t yet get bored. As far as we know. So, that’s beneficial. If I told a human every time I asked it to do something that this was important to my career, like eventually, this prompt would stop working. The LLM doesn’t have that for now.
Brennan Smith
I think this has been a pretty interesting discussion. I think it’s amazing. I mean, still to me, not as a practitioner, I think it’s not obvious that a department of psychology would be doing research like this. But it’s super interesting to think that these incredible innovations that we’ve been watching that have started as chatbots are now in so many other places in our lives and are now going to be. I love the idea that other professions or other disciplines around the world are going to be exploring their use, trying to understand them better, and giving feedback to those CS teams that maybe are not great at typical, I guess, psychological considerations, but that’ll be continuing to improve. What do you think we’ll see in the next year? If you had to make, in closing, what would be your prediction for the next coolest thing that we’re going to learn about an LLM or the next White Paper, we’re going to be like, oh my God. What do you think that’s gonna be?
Mikaela Grace
I think that this world is moving so fast that it’s nearly impossible to tell. I think you’re right on the money that there’s an accessibility here that is new. People being able to talk to artificial intelligence and models using their own language rather than having to write code is going to generate this blossoming of sort of papers and approaches and things that we haven’t thought of yet, because it’s just like you have way more humans able to interact with these models easily. But I don’t think it’s just one specific thing. I think there will be ten white papers in the next year, where we’re like, whoa, crazy.
Brennan Smith
Thank you for listening. We will put in the show notes the paper we’re talking about, a few articles about the paper, and one or two other white papers that we found interesting. We hope that you enjoy them too, and we hope that you’ll tune in to our next episode. Thank you, Mikaela. Welcome to the team.
Mikaela Grace
Thanks Brennan.
Brennan Smith
Thank you listeners. Bye bye.