Why High-Quality AI Data Matters in Global Content
Generative AI continues its relentless market penetration, empowering enterprises to create content for global marketing/localization easily and efficiently. According to Salesforce, 51% of the 1,000 marketers surveyed already use or experimented with AI for work. The favorable data Salesforce revealed about AI isn’t an isolated one. Many AI adoption statistics reveal companies welcome AI into their operations.…
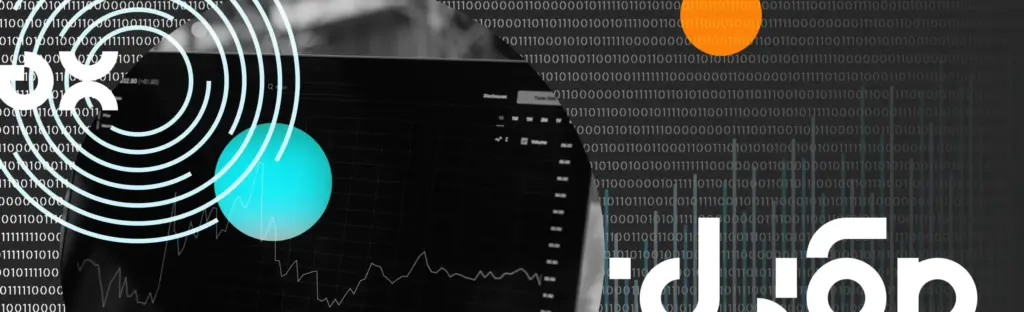
Generative AI continues its relentless market penetration, empowering enterprises to create content for global marketing/localization easily and efficiently.
According to Salesforce, 51% of the 1,000 marketers surveyed already use or experimented with AI for work. The favorable data Salesforce revealed about AI isn’t an isolated one. Many AI adoption statistics reveal companies welcome AI into their operations. Moreover, companies are experiencing excellent results from using AI.
- 79% of leaders reported a decrease in cost due to AI adoption.
- 54% of executives are experiencing increased productivity in their business due to AI.
- 74% of business executives believe generative AI’s benefits outweigh its potential drawbacks.
Considering the benefits AI models can bring to enterprises, the strong acceptance of AI is a logical outcome.
The Benefits of Using AI Models Trained With High-Quality Data
“Quality is never an accident; it’s always the result of intelligent effort. The same principle applies to training AI models. High-quality, multilingual training data is the bedrock of an AI’s system to deliver accurate, effective, and culturally sensitive content that resonates on a global scale.” Kelly Sinclair, Head of Operations, AI Services, Welocalize
AI models developed through high-quality training data can generate safe and reliable AI content. Thanks to AI, what once took weeks or months of content production can now be achieved in days or even hours.
Below are additional noteworthy benefits enterprises seeking global expansion can reap from using AI.
- Increased efficiency
- Multilingual support
- Easier/quicker translation
- Cost reduction
- Improved customer experience
With all the benefits AI brings to global content creation, however, there are risks to consider.
The Dangers of Using Poorly Trained AI Models
AI offers enterprises significant advantages only when trained with high-quality data that has a focus on multilingual data. Using poorly trained AI models can wreak havoc on enterprises looking to connect and engage global/local audiences.
Using inadequately trained AI models can lead to a myriad of issues.
- AI could create culturally insensitive content, which could cause backlash and reputation damage.
- Imprecise word choice or low-quality translation could misrepresent the enterprise’s brand image.
- Enterprises could face legal problems when poorly trained AI models generate content that violates laws on misinformation, copyright, or defamation.
The issues above can be catastrophic to enterprises, which could lead to a brand meltdown, tarnished reputation, and costly fees.
A strong emphasis on using high-quality training data on AI models is an absolute must.
What Is Training Data and Why Do Global Enterprises Need It?
Global enterprises need high-quality training data, especially those focused on multilingual data, to build AI models for global audiences.
Training data is the foundation of how AI models are developed. It refers to the initial data “fed” to the AI algorithm, so it “learns.” By processing the training data, AI algorithms learn structures, patterns, and features, create predictions, perform tasks, and more.
Challenges With AI Data
Obtaining high-quality training data isn’t always easy. Also, enterprises face many obstacles regarding their AI data.
Below are some typical obstacles enterprises face:
- Poor data quality reduces model accuracy. Low-quality training data leads to numerous problems, such as machine learning bias, unreliable projections, and wasted computational resources on retraining. High-quality data collection challenges arise from insufficient data, conflicting data sources, and inefficient data labeling.
- The training data wasn’t gathered with the AI model’s intended purpose and function in mind. Prior to data collection, it’s crucial to set clear objectives for the AI model. This will help you implement measures to ensure data relevance.
- The training data can be challenging to verify. Data validation is another challenging aspect that makes it difficult for organizations to compile high-quality training data. To overcome this, you need a multi-step approach that includes continuous monitoring, data cleansing, and manual review.
- The crowds contributing to the data collection process lack diversity and fail to reflect a broader population or context. Minorities and outliers are sometimes overlooked, especially if the organization doesn’t prioritize data diversity. Unfortunately, ensuring a diverse sample population takes longer and adds new complexity to the data collection process.
Three Pillars of Data Quality: Best Data, Best Models
Exceptional AI models, capable of expanding enterprises’ global reach, rely heavily on high-quality training data.
To give you a clearer picture of what “high-quality data” means, consider these three pillars:
- The training data produced must adhere to established standards and guidelines. It should also align with applicable requirements, internal policies, and industry best practices. When training data is produced with a strong consideration for compliance, the AI model won’t produce legally problematic content or those that pose ethical concerns.
- Diversity plays a crucial role in ensuring the relevance of your training data. The training data must include a wide range of voices, incorporate inputs from various sources and stakeholders, and promote a comprehensive representation of the subject matter while ensuring consistency. When your training data includes irrelevant data points, confusion, and noise are added, which then hurts the efficiency and performance of the AI model.
- Fidelity. This refers to the assurance that the data deliverables are free from fraud, ensuring the information presented is original, accurate, and not misrepresented, enhancing the data’s reliability.
Why High-Quality Data Is the Key to Success
The quality of your training data is pivotal to developing AI models with superior performance. It adds to your AI model’s accuracy, fairness, trustworthiness, and overall performance, all of which can lead to increased productivity and business efficiency.
Sadly, the opposite is also true.
Low-quality training data reduces the overall performance of AI models. It causes AI models to generate inaccurate, faulty, and legally problematic content, resulting in many issues for your enterprise.
Do not settle for low-quality training data.
Obtaining your training data from crowdsourcing platforms, public data sets, user-generated content, or scraping the web can harm your AI model’s performance. These sources are far less ideal since the data obtained isn’t produced with your AI model in mind.
Invest in high-quality training data to springboard your initiatives to leveraging AI modules for your business.
Develop Reliable and High-Performing AI Models
Welocalize helps enterprises train AI models by providing high-quality, multilingual, diverse, and representative training data.
This empowers enterprises to develop reliable AI models capable of generating high-quality translations, localized marketing materials, multilingual content, or most forms of content that enable global reach.
Reach out to Welocalize now for help training your AI models with high-quality, multilingual data.