Building AI We Can Trust, Ethical Non-Bias Machine Learning Makes for a Safer Internet
Artificial intelligence (AI) and data are pivotal in shaping our online experiences in today’s digital age. AI algorithms, from search engines to content recommendations, are constantly at work, influencing the information people encounter online. However, harnessing the power of AI comes with great responsibility. You must create data sets to drive performant large language models (LLMs) and…
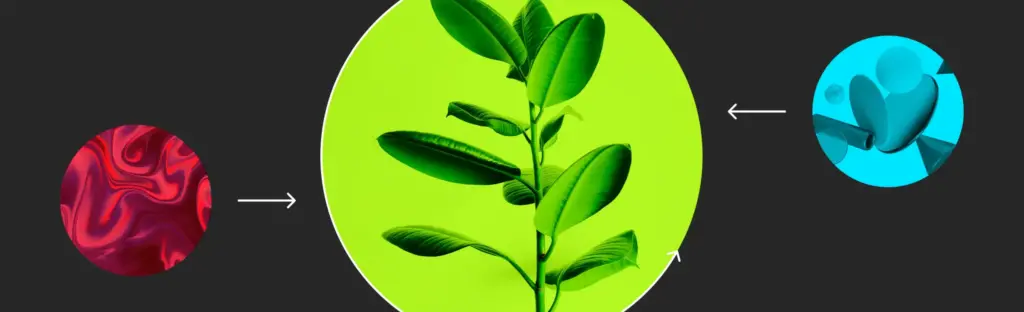
Artificial intelligence (AI) and data are pivotal in shaping our online experiences in today’s digital age. AI algorithms, from search engines to content recommendations, are constantly at work, influencing the information people encounter online. However, harnessing the power of AI comes with great responsibility.
You must create data sets to drive performant large language models (LLMs) and AI models to get great, accurate results. Using and creating reliable data sets also ensures your AI models have minimal hallucinations and safe, non-biased output, whatever the language.
This guide covers the importance of leveraging ethical, non-biased machine learning (ML) to ensure trust in AI systems and outputs for a safer internet for all users.
The Role of Machine Learning in AI
Machine learning is vital to AI models (including generative AI models like ChatGPT and Bard). ML trains the AI agent on a particular function by running billions of calculations and learning from them. The whole task is faster than human training due to its automation.
ML is crucial for developing models and algorithms that can learn and make decisions or predictions without explicit programming. ML is vital for:
- Ensuring model fairness. Machine learning can ensure fairness in models by mitigating bias in AI algorithms and training data. It helps reduce algorithmic bias in AI.
- Data-driven learning. ML is essential for training AI models by exposing them to diverse, and representative data sets that have been curated to avoid incorporating unfair practices and reinforcing generative AI bias.
- Guarding against discrimination. Incorporating ethical considerations into AI model design and training (via ML) helps avoid amplifying existing social biases in the AI system.
The Impact of Data Quality
A foundational pillar of reliable AI is high-quality data. After all, the effectiveness of AI models depends heavily on the data used to train them. It makes data quality critical to ensure trustworthy, non-biased ML for building AI systems that help make the internet safer. AI models built from top-quality training data can result in reliable and safe AI content while streamlining content production and translation and reducing costs.
Filtering Harmful Content
The quality of the data used for training AI models impacts the models’ ability to spot and filter harmful content. For example, using training data sets with harmful and safe content examples helps ML models differentiate the two based on features within the data. It can help protect users from harmful information since you can train the models to filter potentially harmful links and content from search engine results pages.
Ethical Machine Learning
AI models aren’t inherently biased or toxic; however, they can reflect the biases in the data they are trained on. To create trustworthy AI, you must address bias and toxicity issues in machine learning and the training data, including the following.
Gender Bias in AI
Historical data, whether in the education, employment, or healthcare sector, can reflect societal gender stereotypes, resulting in AI gender bias. One of the common examples of bias in AI is including stereotypes in the data for training models, resulting in the models learning, perpetuating, and reproducing them in their outputs and predictions.
An effective approach to mitigate this is to use representative and diverse training data that includes a broad range of experiences and perspectives. Opt for a balanced data set across race, gender, age, and other crucial demographic factors to ensure the AI model learns from comprehensive examples. It can reduce potential issues such as AI racial bias.
It lowers the chances of using bias in AI examples, such as training data, that leads to AI bias in healthcare, hiring, and other industries and sectors. AI models can also amplify stereotypes. For example, let’s suppose natural language processing (NLP) models use training data containing biased examples or language. In that case, they can learn to associate specific occupations or traits with particular genders, resulting in biased outputs.
The key is implementing techniques and tools to catch and assess biases and stereotypes within the training data and the AI model’s predictions. You can track and analyze fairness indicators and metrics throughout the model’s lifecycle to examine how gender stereotypes influence the model’s outputs.
Toxicity and Hate Speech
AI models can inadvertently generate toxic or hate speech. The right data and machine learning techniques can prevent this and promote healthy online user interactions. Here’s how:
Challenge #1
Context misinterpretation. AI models can struggle to understand nuanced language contexts, causing them to generate offensive or inappropriate language and resulting in bias and discrimination in AI.
Solution
Develop models that understand the context within nuanced language to learn how to reduce bias in AI and potential misinterpretations while promoting respectful, contextually accurate output.
Challenge #2
Adversarial vulnerability. AI models can be vulnerable to adversarial attacks where data is intentionally manipulated, causing the models to generate harmful or toxic content.
Solution
Train AI models to safeguard against intentional manipulations via adversarial training. It can keep the models robust and resistant to manipulations, reducing the risks of generating toxic or hateful content.
Challenge #3
False negatives and positives. AI models don’t always accurately distinguish harmful from non-harmful content, leading to false negatives or positives when filtering content.
Solution
Implement solid systems for users to provide feedback and report toxic content to help facilitate continuous improvement. Also, prioritize adherence to ethical guidelines when developing AI models to ensure responsible and unbiased practices.
The best ways to address the challenges that lead AI models to generate toxic or hate speech include implementing model development strategies, data curation, and ongoing monitoring and analysis.
Leverage Machine Learning for Trustworthy AI and a Safer Internet
Building AI we can trust goes beyond being mindful of the algorithms; it’s also about the data and machine learning processes that underpin them.
Ensuring data quality, structuring data effectively, and implementing ethical machine learning practices help you make significant strides toward a safer internet environment with accurate search results, filtered harmful content, and minimal AI bias and toxicity.
This guide underscores the critical role of data and ethical machine learning in creating an internet that’s a reliable and secure resource for all users.
Welocalize has used AI innovation for decades, from neural machine translation and NLP to developing multilingual chatbots and fine-tuning LLMs.
We keep “international” at the heart of what we do.