Customer Support Translation: Localizing Your Knowledge Base
Support content is a crucial part of the customer experience. Learn how you can save time and money on the localization of knowledge base materials with machine translation and large language models.
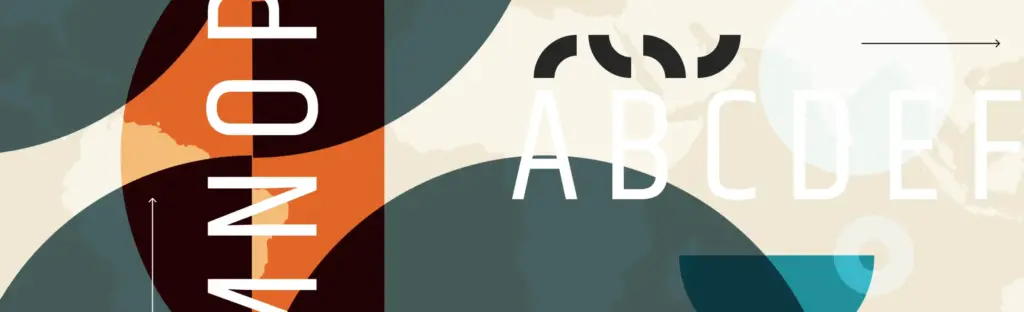
Support content is a crucial part of the customer experience. A survey conducted by CX Network showed that nearly two-thirds of consumers prefer self-service digital channels over phone calls and store visits to resolve issues, with FAQs as the most popular tool. And the long-running CSA Research study highlighted that 76% of consumers worldwide prefer buying products with information in their language.
Localizing your support content, such as FAQs and knowledge base articles, is a must for your business to grow internationally. Users all over the world need to understand them. If your support content is only available in one language, you miss out on an enormous opportunity to reach a global audience.
However, support content is often high volume, constantly changing, and technical. Knowledge base translations must be accurate, consistent, and frequently updated. However, this comes at a price: translating knowledge articles can be time-consuming and costly.
This is where machine translation (MT) comes in. Neural machine translation (NMT) combined with human post-editing has made it possible to localize support content faster and more efficiently. With advances in artificial intelligence (AI) and large language models (LLMs), the speed and efficiency of support content localization are expected to accelerate further.
Machine Translation for Knowledge Base Translation
There are two ways to translate support content: manual translation using human translators and linguists or MT using human post-editing. When highly trained, MT can be as effective as human translation when post-edited.
Using MT to automatically translate text from one language to another is more cost-effective with faster turnaround times. Particularly if your users simply need to gain a basic understanding of the content and, therefore, little or no post-editing is required. As your support content volume increases, with MT, you won’t need a proportional increase in resources.
NMT, which uses deep learning algorithms to learn from large amounts of data, produces more natural, accurate, and fluent translations.
MT can be customized and trained to suit a specific domain, terminology, and style preference. At Welocalize, as well as using generic MT engines, we develop highly trained custom MT engines using a company’s existing translation memories (TMs), glossaries, and style guides. This allows us to scale knowledge base localization faster and with cost savings.
“Knowledge base articles are often one of the first content types our clients select for their MT workflows. To customize your machine translation model, you’ll need a dataset of knowledge base articles in both the source and target languages. This dataset should be representative of the content you want to translate and include your domain-specific terminology and style. Ideally, your dataset will include thousands of articles to ensure that the machine translation model can learn the nuances of your content effectively. However, with the help of LLMs, we are able to customize with smaller data sizes, and a dataset of a hundred translated articles plus a glossary is a good starting point.” Elaine O’Curran, Senior AI Program Manager at Welocalize.
Training MT engines is only part of the equation. LSPs must combine MT with human post-editing and quality evaluation to fix errors and ensure translations meet quality requirements.
“By closely monitoring user engagement metrics and making data-driven adjustments to your post-editing process, you can optimize the quality and effectiveness of your translated knowledge base content by ensuring that your users receive the support they need, regardless of their language,” O’Curran shared. “For example, if machine-translated articles in a specific language consistently receive low satisfaction ratings or high bounce rates, consider adding light or medium post-editing for that language to improve translation quality.”
Large Language Models: The Future of FAQ Localization
LLMs represent the next frontier in content generation and translation. The rapid rise of ChatGPT, Gemini, and other generative AI that can automatically create and translate content has caused a lot of commotion in the language services industry.
LLMs are a new generative AI model that can produce natural language text based on a given input or prompt. Trained on massive amounts of text data from various sources and domains, LLMs can generate cohesive, relevant, and natural-sounding content from scratch.
The potential use cases in translation and localization are also very promising. For example, companies can use LLMs to generate new content in different languages based on existing content in one language. This can help brands create multilingual support content faster, easier, and cheaper.
However, LLMs are still at a nascent stage and not yet ready to fully replace MT or human translators. AI-generated and translated content can be inaccurate, biased, or inappropriate for your target audience.
Welocalize conducted a study comparing the performance of several LLMs vs. custom MT on the quality of translations for customer support content. Results showed that custom NMT models outperformed LLMs and combined NMT-LLM models. LLMs like GPT-4 cannot yet match the performance of highly trained NMT engines. However, they came very close to meeting quality standards.
Still, LLM models designed to translate content for specific contexts, domains, tasks, or customer requirements must be fine-tuned. They need custom training data to improve their ability to provide more accurate translations for different use cases.
The role of LSPs is, therefore, expected to change, focusing on creating training data to make LLMs more accurate, domain-specific, and customized to each company’s needs.
AI-Enabled Quality Evaluation for Support Content
One area where AI already stands out is AI-enabled quality evaluation (AIQE). This approach to measure and improve the quality of translated and localized content uses AI and machine learning (ML). It’s a viable alternative to human post-editing or review, which saves time and cost.
AIQE uses quality metrics and indicators, such as fluency, adequacy, accuracy, consistency, terminology, style, and more, to evaluate the quality of translations produced by MT engines or LLMs. AIQE also provides valuable feedback and suggestions on the quality of your translations and the performance of your MT engines or LLMs.
The main difference between human reviewers is everything is automated. AIQE tools can automatically assess the quality of translations or generated content, flagging potential issues, such as mistranslations, inconsistencies, or readability problems. AIQE can also ensure your support content remains consistent with your company’s terminology and glossary, reducing the risk of confusing or misleading translations.
Because of automation, quality control is more scalable. Even if the volume of support content skyrockets, AIQE can scale alongside it without requiring an army of human reviewers.
Work With Welocalize
Welocalize offers various solutions to help you localize your support content and knowledge bases using the latest innovations. As a pioneer in using AI to translate multilingual content, Welocalize is at the forefront of generative AI in language services. We have redesigned our workflows, embedding LLMs and developing new techniques to meet the demand for translating AI-generated content.
Contact us today if you want to learn more about how Welocalize can help you localize your support content.