Building Trust in AI: Key Strategies for Ensuring AI Data Integrity and Quality
Discover strategies for ensuring AI data integrity in AI training, including workforce vetting, fraud detection, and mitigating bias for reliable AI models.
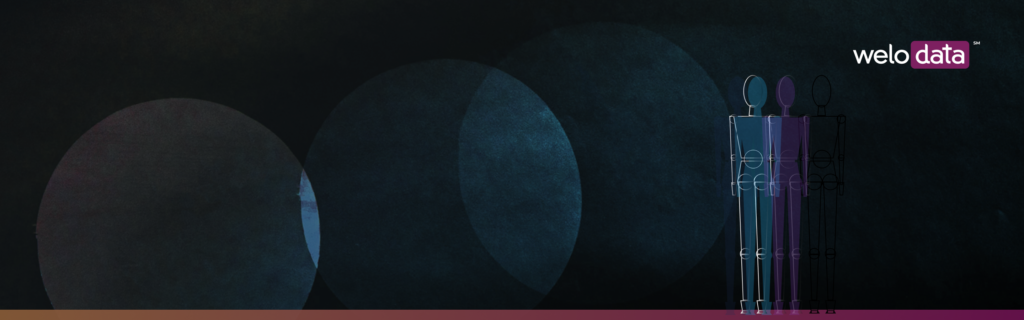
As artificial intelligence continues to evolve, the integrity of the data used to train AI models has become increasingly critical. Ensuring the trustworthiness and quality of this data is essential to building AI models that perform reliably and accurately across industries such as healthcare, finance, and technology. Data integrity, workforce vetting, and fraud prevention are now central to developing robust AI systems, especially as these models are used to make impactful decisions.
During a recent webinar, Trustworthy AI: Technologies and Strategies for Data Integrity, hosted by Welo Data, industry experts discussed strategies to ensure data integrity in AI training pipelines. They explored challenges such as verifying workforce qualifications, preventing fraud, and maintaining high data quality throughout the training process.
As Sarha Mavrakis, Head of Commercial at Welo Data, emphasized, “Data integrity is the backbone of any successful AI model. Without it, even the most advanced algorithms can produce biased or inaccurate results.” This article outlines key strategies from the webinar to help businesses build reliable AI systems with trustworthy data.
Sarha Mavrakis, Head of Commercial, Welo Data“Data integrity is the backbone of any successful AI model. Without it, even the most advanced algorithms can produce biased or inaccurate results.”
Data Integrity: The Foundation of Reliable AI
In the AI development process, data integrity plays a crucial role in ensuring that models perform as expected. The quality of the data used to train AI models directly influences the accuracy and reliability of the system. Any inaccuracies, inconsistencies, or biases in the training data can lead to flawed AI outputs, which may have significant negative impacts on real-world applications.
Welo Data, a leader in AI training data solutions, has developed tools like NIMO, a network identity management and operations platform, to tackle challenges related to data quality and verification. By focusing on data origin and trust, NIMO helps businesses maintain higher standards of data integrity, ensuring that their AI models are reliable and free from contamination.
Workforce Vetting: The First Line of Defense for AI Data Integrity
One of the first steps in ensuring data integrity is carefully vetting the workforce responsible for preparing and labeling the data. AI models depend on the quality of the data they are trained on, which means that contributors must be skilled and trustworthy.
Canute Haroldson, Head of Growth Strategy at Welo Data, emphasized the value of workforce vetting. “You want to be able to do identity verification, and, really importantly, you want to be able to do that in concert with your onboarding,” he said. The process involves verifying the credentials and backgrounds of contributors, including checking government-issued documentation, certifications, and relevant work experience. This helps prevent individuals with false qualifications from entering the system.
Canute Haroldson, Head of Growth Strategy, Welo Data“You want to be able to do identity verification, and, really importantly, you want to be able to do that in concert with your onboarding…”
Vetting is not limited to the onboarding process. Ongoing monitoring of the workforce is essential to maintaining data quality throughout the project lifecycle. Carrie Campbell, Head of Data Quality at Welo Data, pointed out, “We have to continually verify that the person continues to be who they say they are, where they say they are.” This ongoing verification ensures that contributors maintain the required standards of quality and integrity.
Preventing Fraud in AI Data Preparation
Fraud in AI data training can seriously undermine the accuracy of AI models. Fraudulent activities—such as misrepresenting identity, spam accounts, or exam cheating—can introduce compromised data into the training process, leading to inaccurate AI models. Detecting and preventing fraud early is key to maintaining AI data integrity.
Welo Data uses advanced tools to detect potential fraud, including biometric verification methods such as facial recognition and fingerprint scanning. These technologies help ensure that contributors are who they claim to be. Physical characteristics can also be used to verify digital profiles, ensuring that the person behind the screen is legitimate.
Additionally, machine learning algorithms can be used to flag suspicious patterns in contributor behavior, such as rapid job changes or abnormal activity during annotation tasks. “We track and check for the endless ways that people are trying to game systems, and we have to do all this while scaling and managing quality and programs that have rapid deployments,” Campbell added, underscoring the importance of evolving strategies to stay ahead of fraudulent actors.
Carrie Campbell, Head of Data Quality, Welo Data“We track and check for the endless ways that people are trying to game systems, and we have to do all this while scaling and managing quality and programs that have rapid deployments…”
By implementing these multi-layered fraud detection techniques, businesses can prevent unreliable data from entering their AI training pipelines, protecting the integrity of their models.
Scaling AI Data Training Without Compromising Quality
As AI adoption accelerates across industries, many companies face the challenge of scaling their AI training teams quickly without sacrificing data quality. Rapid scaling often requires onboarding large teams of data annotators within short timeframes, which can strain quality control processes.
Haroldson highlighted the need for automation in scaling AI data training. “You can actually utilize the technology to streamline and automate the onboarding process and make it pretty continuous. So, you can utilize AI-driven platforms to personalize learning paths,” he said. Welo Data’s approach to scaling involves creating modular, role-specific training content that helps contributors ramp up quickly and efficiently.
Automating parts of the onboarding and training processes ensures consistency across large teams while maintaining data integrity. Aptitude needs to be screened, and contributors must be ready to produce high-quality data from day one. This ensures that contributors are equipped to handle the specific tasks they are assigned, even in fast-paced, high-growth projects.
Real-time performance tracking is also essential to maintaining quality during rapid scaling. Continuous monitoring helps identify issues early, allowing businesses to address any problems before they impact the AI model’s performance.
Cultural Competency: Addressing Global AI Data Challenges
As AI systems are deployed in global markets, cultural competency becomes increasingly important in ensuring accurate data. Contributors working on data annotation and labeling must understand the cultural nuances of the regions they are representing. Without this understanding, they risk misinterpreting data, which can lead to biased or inaccurate AI models.
During the webinar, Haroldson discussed the importance of cultural competency in AI data preparation. “Provide ongoing cultural training support to make sure that contributors are well equipped to handle data that has cultural sensitivity and also deliver that accurately,” he said. For example, a Spanish speaker from Ecuador may interpret certain data differently than a Spanish speaker from Spain. These distinctions must be accounted for in the data training process to avoid skewing AI outputs.
“Provide ongoing cultural training support to make sure that contributors are well equipped to handle data that has cultural sensitivity and also deliver that accurately…”
Canute Haroldson, Head of Growth Strategy, Welo Data
To address this, Welo Data includes cultural competency assessments as part of its vetting process. Contributors are selected based on their familiarity with the cultural context of the data they will be working with. In cases where cultural nuance is critical, such as in localized content or region-specific tasks, contributors with lived experience in the relevant locale are prioritized.
Mitigating Bias in AI Models Through Diverse Data Pools
Preventing bias in AI models is one of the most important tasks in AI development. If training data reflects bias—whether intentional or unintentional—AI models will replicate and amplify that bias in their results, leading to potentially harmful outcomes.
A key strategy for mitigating bias is ensuring that the data pool used for training is diverse and representative of the intended population. By selecting contributors from diverse demographic backgrounds, companies can reduce the likelihood of training data being skewed toward one particular group.
Bias prevention also involves ongoing monitoring and regular audits of the AI model’s performance to ensure that the data remains balanced and free from bias throughout the training process. By addressing bias proactively, companies can ensure that their AI models are fair, ethical, and capable of serving a broad range of users.
Data Integrity as the Key to Trustworthy AI
It is clear that AI data integrity is the foundation of reliable AI models. To build AI systems that businesses and consumers can trust, companies must prioritize strategies that protect the quality and authenticity of their training data.
From thorough workforce vetting and fraud detection to cultural competency assessments and bias mitigation, these strategies are essential for ensuring data integrity throughout the AI development process. By adopting best practices and leveraging advanced technologies, businesses can create AI models that are not only high-performing but also fair, ethical, and trustworthy.
Data quality is driven by data integrity, and you cannot have one without the other. By focusing on both, companies can ensure that their AI models deliver accurate, reliable, and impactful results.
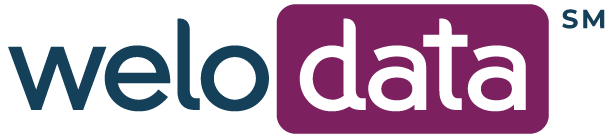