Insights
if ( ! empty( $excerpt ) ) :?>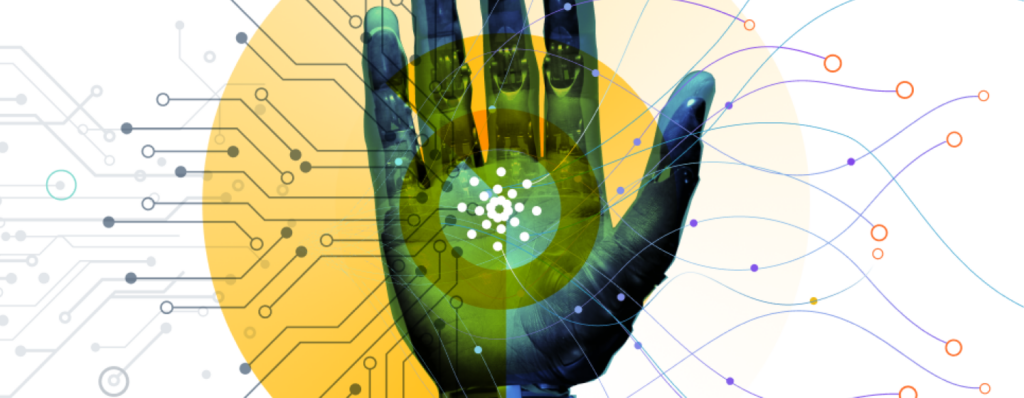
Featured Insights
Is your business ready to scale with the latest technologies? Catch up on industry news and see what experts have to say.
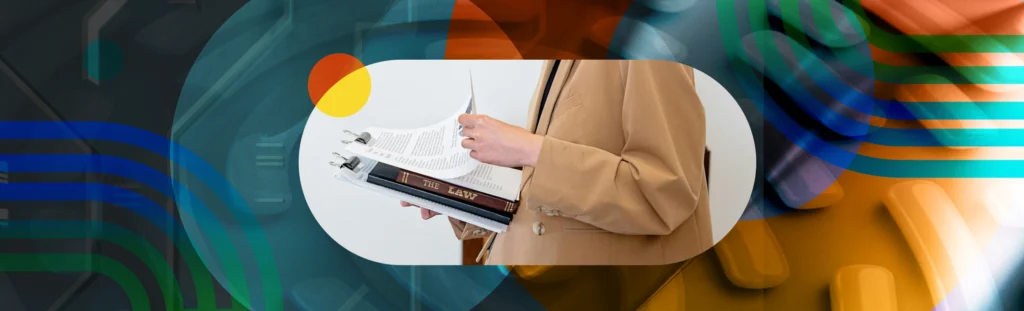
Navigating Global Patent Filing in 2025: Trends, Challenges, and Solutions
Emerging technologies, including specialized language tools and blockchain, are reshaping…
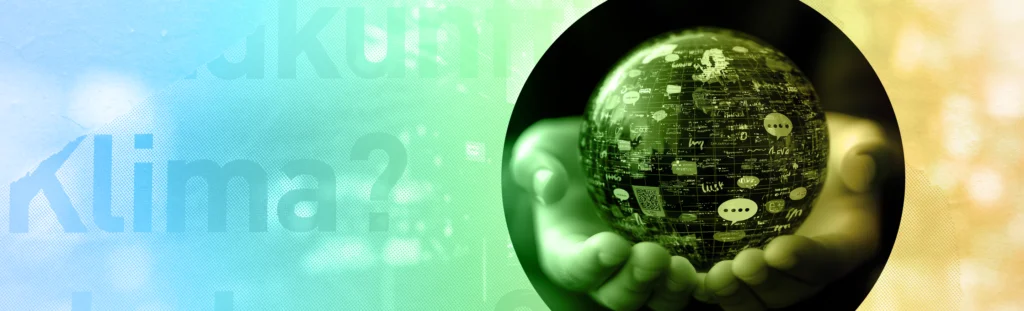
Rethinking Accessibility in Live Events: Practical Insights for Inclusive, Multilingual Experiences
Explore strategies to ensure accessibility and multilingual inclusion for hybrid…
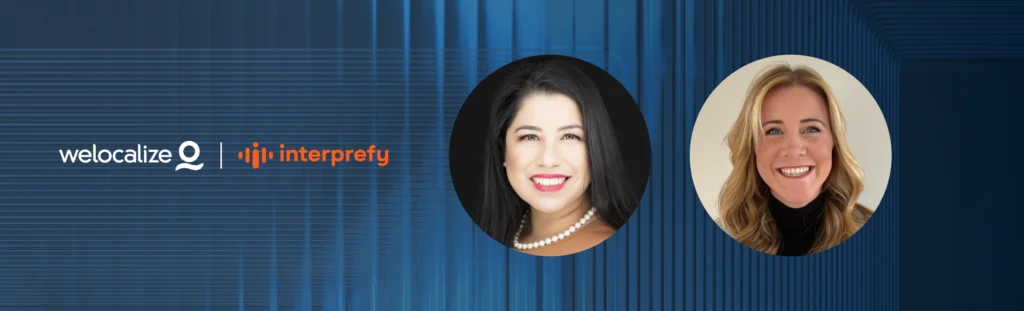
[On-Demand] Rethinking Accessibility In Live Events
Watch on-demand and explore effective strategies and technologies for improving…
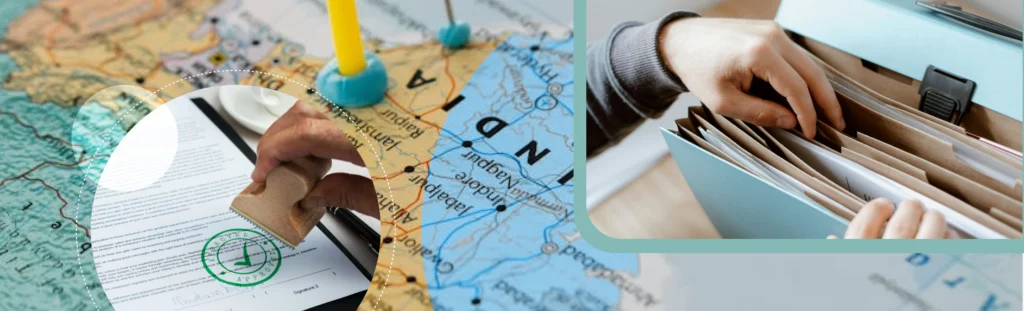
The Rise of Patent Filings in India and What It Means for Innovation
India is emerging as a global innovation leader, currently ranking…
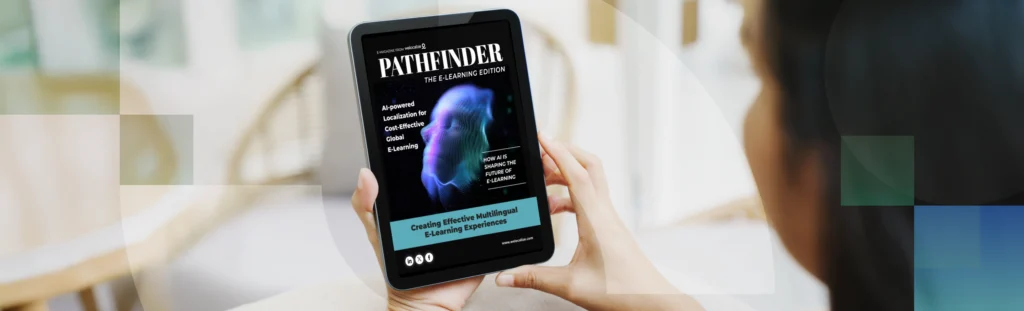
PATHFINDER | The E-Learning Edition #2
Welcome to the second e-learning edition of PATHFINDER. In this…
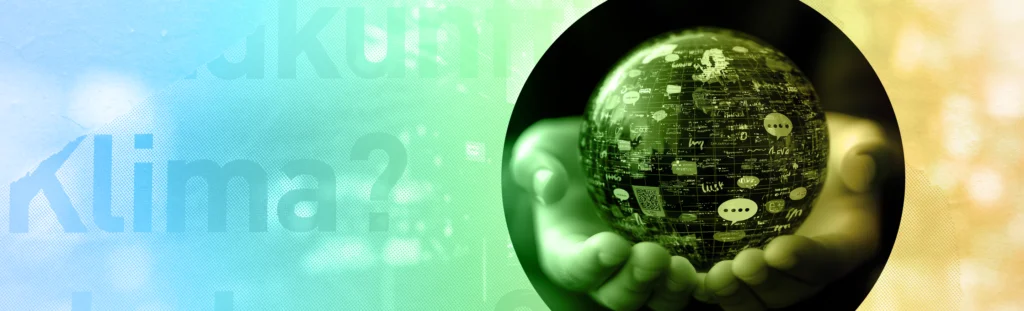
CASE STUDY: AI-Powered Multilingual Subtitling in 3 Days
Learn how a global technology leader achieved fast, accurate multilingual…
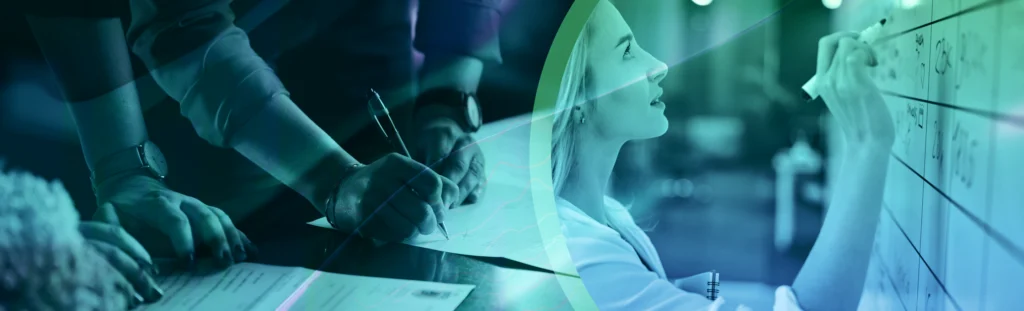
Legal Operations Control: Optimizing Multilingual Workflows for Efficiency and Accuracy
With the new administration in place, today’s in-house legal teams…
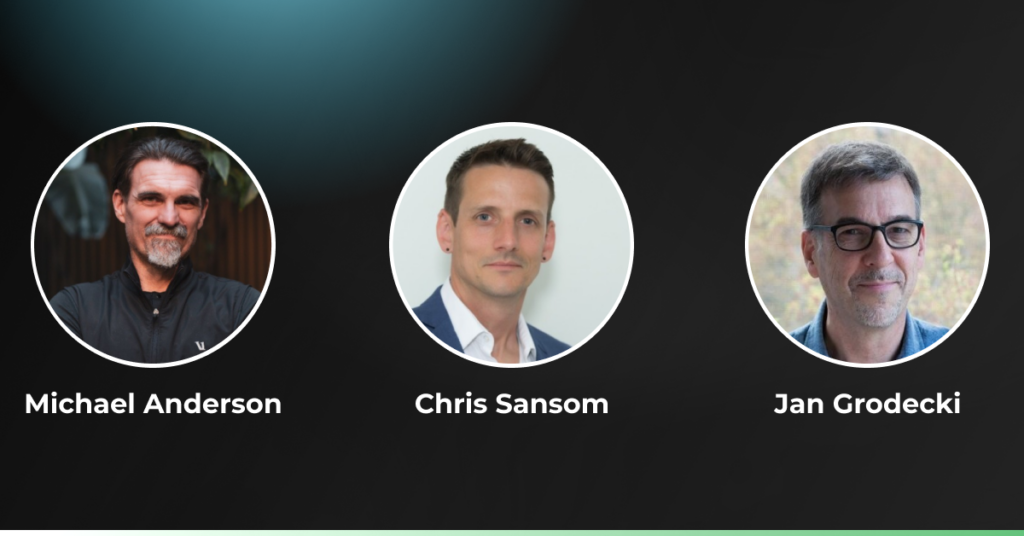
AI-Driven Innovation: Redefining E-Learning and Localization for a Global Audience
Explore how AI tools are optimizing e-learning localization, enhancing content…
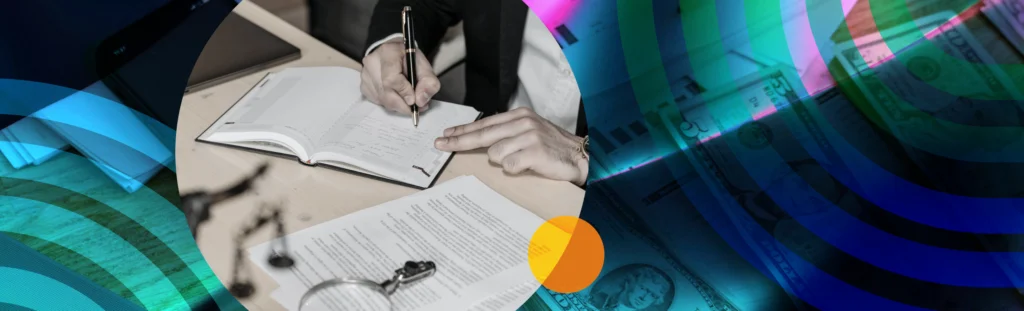
Efficient IP Filings: Reducing Risk and Accelerating Global Prosecution
Discover strategies to optimize global patent filing for efficiency, reduce…
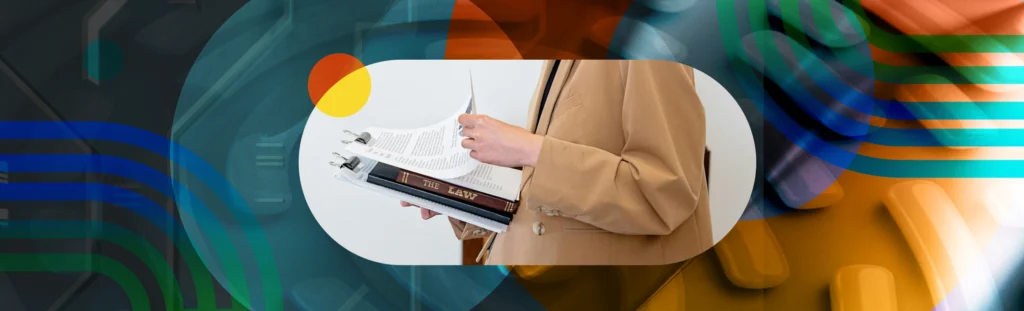
Navigating Global Patent Filing in 2025: Trends, Challenges, and Solutions
Emerging technologies, including specialized language tools and blockchain, are reshaping…
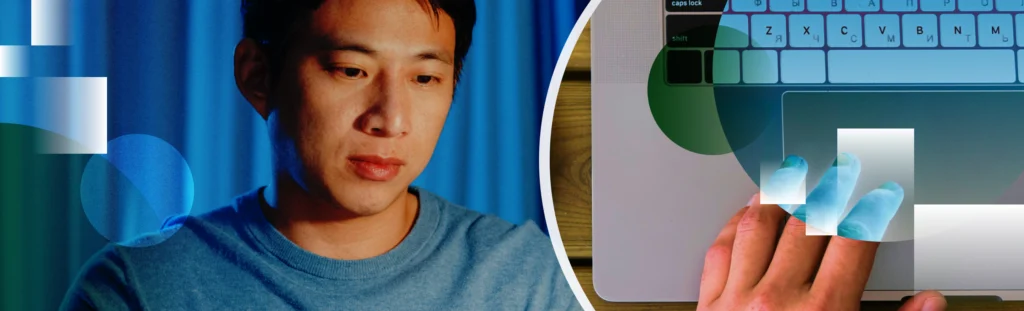
CASE STUDY: Streamlining Training Video Localization for a Global SaaS Platform
Delivering multilingual training content with localized UI, voiceovers, and 300+…