The Pros and Cons of Using AI for Multinational Cases | Chapter 2
Applying AI to E-Discovery Translation
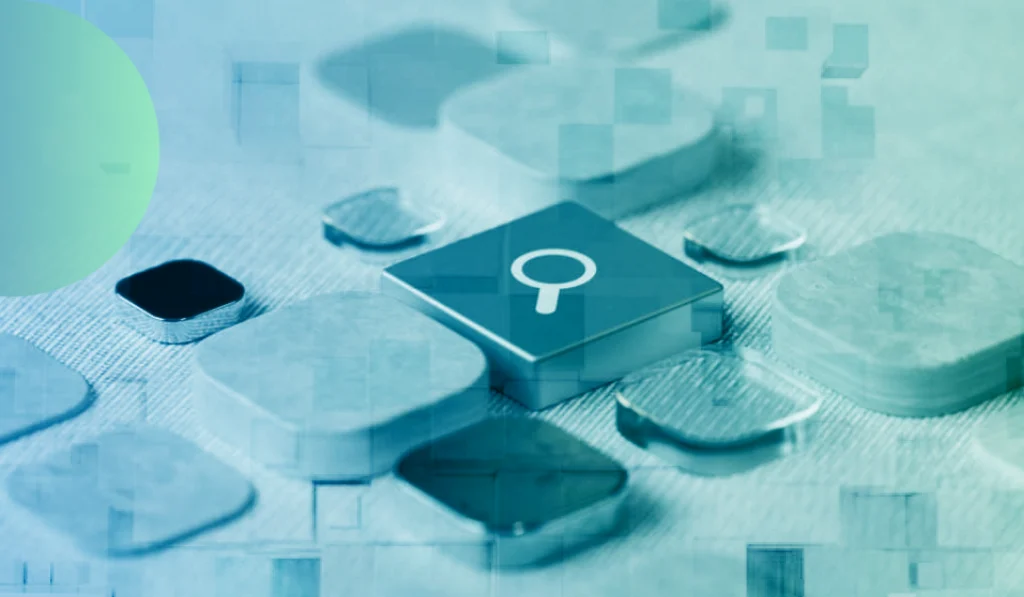
AI and GenAI present massive opportunities in litigation, as well as several new challenges.
Understanding where and how different AI technologies can help or hinder eDiscovery is essential.
Machine translation has become more common in e-discovery in recent years because of its efficiency.
GenAI has the potential to be a very powerful e-discovery translation tool for cross-border litigation.
Many are turning to a hybrid approach that combines AI and human intervention to improve the efficiency of e-discovery.
AI is here to stay. However, legal teams must still include sector-specific linguists to handle nuanced translations.
During e-discovery, legal teams search, secure, and utilize electronic data as legal evidence. When the process involves multiple languages, it becomes complex, costly, and incredibly time-consuming to identify, read, and certify relevant documents.
Poor translations or missed deadlines can also have serious consequences, such as heavy fines, investigations, and legal liability. Legal discovery teams need to be quick, but they also need to be right.
In recent times, translation during e-discovery has been conducted exclusively by humans, mostly by subject matter experts who understand intricate jurisdictional requirements—including details like paper size, how to structure claims, how to number paragraphs, and more—as well as key linguistic nuances in the actual body text.
These professionals ensure that a source document reads the same way in France as it does in Brazil or China so that there’s no room for interpretation.
Machine Translation in E-Discovery
Machine translation (MT), developed and refined before the advent of GenAI, has become more common in e-discovery in recent years because of its efficiency.
Translating large numbers of documents collected during e-discovery is time-consuming and very expensive. However, while machine translation can be used to identify the content of a document in broad terms, MT outputs are the most reliable when performed in conjunction with a human counterpart.
Machine translations are very literal, based on a set of rules or patterns, and can generate errors in e-discovery translation. This type of translation demands heightened attention to linguistic, cultural, and situational nuances, in addition to jurisdictional formatting requirements.
As a result, standard MT engines available within e-discovery platforms like OpenText and Relativity often fail to meet e-discovery localization and translation standards when they’re not paired with human oversight and expertise.
Today, traditional MT technologies are best used during e-discovery for identifying what languages are present in larger data sets and organizing them accordingly, conducting advanced text searches for key terms, and for enhanced optical character recognition, which extracts text from both scanned documents and audio files so multilingual data can be processed at scale.