The Pros and Cons of Using AI for Multinational Cases | Chapter 1
AI vs. GenAI in E-Discovery: The Risks and Rewards
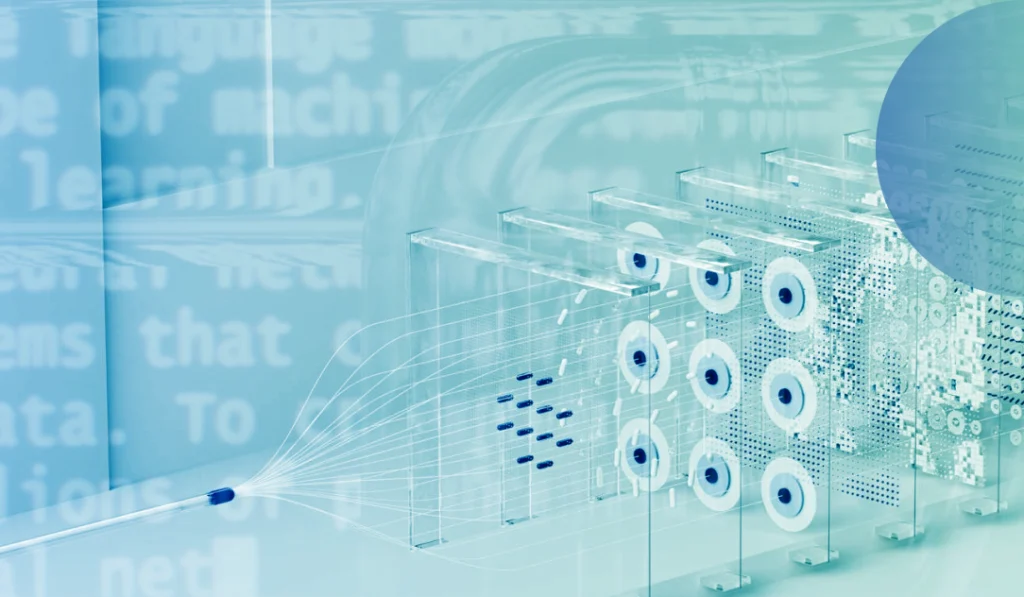
AI and GenAI present massive opportunities in litigation, as well as several new challenges.
Understanding where and how different AI technologies can help or hinder eDiscovery is essential.
Machine translation has become more common in e-discovery in recent years because of its efficiency.
GenAI has the potential to be a very powerful e-discovery translation tool for cross-border litigation.
Many are turning to a hybrid approach that combines AI and human intervention to improve the efficiency of e-discovery.
AI is here to stay. However, legal teams must still include sector-specific linguists to handle nuanced translations.
It’s important to understand where and how different technologies can help or hinder e-discovery. Traditional AI in the context of e-discovery is built on rule-based machines or statistical engines that are fed small amounts of contextual data relevant to a case. It seeks to understand the data and its rules and make accurate predictions strictly from patterns in that data set.
Traditional AI allows legal professionals to gather insights from large sets of data and hone in on the information that matters most, resulting in greater efficiency, more time for strategic thinking, and, ultimately, more value delivered to clients.
Technology-Assisted Review (TAR)
The most common use case of traditional AI in litigation is technology-assisted review (TAR), also known as predictive coding. TAR is a popular software tool that legal teams use to review documents during the discovery process in litigation.
TAR uses machine learning algorithms to classify documents based on review input, which can help teams:
- Prioritize and find relevant documents
- Complete projects faster
- Save costs for clients
- Identify potential case merits
- Structure a case
- Prepare for depositions, motions practice, and trial
Through machine learning, TAR’s predictive algorithms can also transform risk assessment and case strategies. Historical case data can now provide insights to aid litigators in determining the value of contingency-based cases, managing client expectations, and guiding strategic decisions. TAR’s defensibility in court is now well-established and supported by over 10 years of legal precedent.
Generative AI (GenAI)
GenAI, on the other hand, is an emerging technology trained on massive data sets that are not confined to case-specific data. Unlike TAR, GenAI is designed to access, scrape, and process massive amounts of data, allowing it to generate new and novel content, including text, video, and other forms of media.
Large language models (LLMs), like OpenAI’s ChatGPT and Google’s Gemini, have emerged as the most popular Gen AI models, but today many law firms and legal departments are building their own proprietary LLMs for legal-specific use cases.
While LLMs also have the capacity to learn, they are built on neural networks, which function more like a human brain, taking into account the entire context of a given input. As a result, they can generate outputs that are more statistically accurate and situationally accurate for tone, historical context, and cultural nuances.
However, because it’s built on widespread data scraping, GenAI is not yet defensible in court, and opens up legal teams to liability in the form of copyright infringement, confidentiality issues, ethical concerns (outputs may be biased), and inaccuracies (GenAI has a tendency to hallucinate).
For example, in May 2023, during a trial involving a personal injury claim brought against Avianca Airlines, the claimant’s lawyers used ChatGPT to draft legal submissions, which generated case authorities (in addition to quotes and citations) that were fictitious.
Down the line, once data input into LLMs and the quality of their output improves, GenAI is slated to become a powerful legal tool (particularly for e-discovery) and, theoretically, more defensible in court.