The Power of Retrieval Augmented Generation (RAG) in GenAI & Global Content
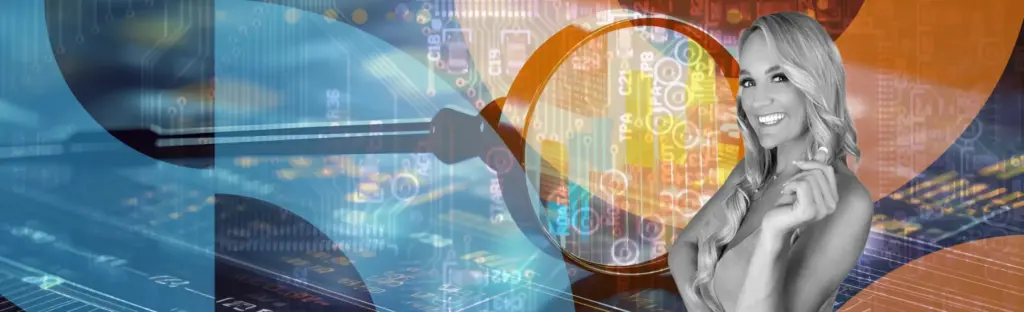
In this guest post, Welocalize AI specialist Tiarne Hawkins delves deep into the world of Retrieval-Augmented Generation (RAG), its impact on Generative AI performance, and other pivotal data concepts.
In the ever-evolving landscape of artificial intelligence (AI), understanding data concepts is not just important—it’s imperative. These concepts are the bedrock upon which AI advancements are built, shaping technologies that redefine our interaction with digital global content. One such groundbreaking development is RAG, one of the latest advancements in AI and language processing.
RAG is an effective AI framework to provide relevant data as context for generative AI (GenAI) models – improving the quality and accuracy of GenAI and LLM output.
So, let’s look at some of the RAG basics and why you should be thinking about RAG for global AI models…
What is RAG?
Retrieval Augmented Generation (RAG) is an advanced AI framework used to enhance the capabilities of large language models (LLMs). At its core, RAG integrates three key elements: retrieval, augmentation, and generation. This process can be likened to a highly efficient librarian who retrieves relevant and timely information from a vast knowledge base, which is then used to augment and inform the responses of language models.
RAG represents a blend of traditional language models with the dynamic retrieval of external data, marking a significant evolution in natural language processing (NLP). This innovation emerged as an answer to the constraints faced by early NLP models. It has enhanced AI’s proficiency in generating responses that are not only accurate but also rich in context and detail, thus redefining the standards of AI-driven language understanding and creation.
Significance of RAG in NLP
RAG’s impact on NLP is profound. It has revolutionized how AI systems interact, understand, and generate human language. With use cases ranging from sophisticated chatbots to complex content creation tools, RAG has been pivotal in improving language models, making them more versatile and intelligent. It bridges the gap between the static knowledge of traditional models and the dynamic, ever-changing nature of human language.
Key Components & Benefits in Global Content & GenAI
RAG combines the depth of language models with the breadth of external data retrieval, transforming global content creation. It allows for AI-driven content that is both linguistically rich and globally informed, breaking down barriers and enabling culturally nuanced communication. In the GenAI era, RAG’s influence is unmistakable, offering a new paradigm in content creation that is dynamic, informed, and universally relevant.
Key Components of RAG
- Hybrid Model Architecture: RAG combines traditional language models with a retrieval mechanism. This hybrid structure allows it to generate responses based on learned patterns and fetch relevant information from external databases or the internet in real-time.
- Dynamic Data Retrieval: RAG can dynamically access many external data sources. This feature lets it pull in the most current and relevant information, making its responses more accurate and up-to-date.
- Deep Learning and NLP Integration: At its core, RAG integrates advanced deep learning techniques with sophisticated natural language processing. This integration allows a deeper understanding of language nuances, context, and semantics.
Benefits in Global Content and GenAI World
- Enhanced Accuracy and Relevance: RAG’s ability to enhance its responses with external data ensures that the information it generates, or processes is accurate and highly relevant to the current context and global developments.
- Cultural and Linguistic Nuance: Understanding and respecting cultural and linguistic nuances is crucial in global content creation. RAG’s advanced NLP capabilities, combined with its access to diverse data sources, enable it to tailor culturally sensitive and linguistically appropriate content.
- Real-Time Information Integration: RAG’s real-time data retrieval capability is invaluable in an era where information constantly evolves. It allows AI systems to incorporate the latest news from around the globe, keeping the content fresh and relevant.
- Scalability and Versatility: RAG models are highly scalable and versatile, making them suitable for various applications in content generation, from automated news reporting to personalized content recommendations. This versatility is particularly beneficial in the GenAI world, where adaptability to different types of content and audiences is vital.
- Breaking Language Barriers: Breaking language barriers is essential for global content. RAG can assist in translating and localizing content, making it accessible to a broader audience while maintaining the integrity and context of the original information.
- Informed Decision Making: By providing more contextually rich and informed content, RAG aids in better decision-making processes in various sectors like business, healthcare, and education, where understanding global contexts and nuances is crucial.
Why Use RAG?
As can be seen above, the adoption of RAG in AI applications offers numerous benefits. It enhances accuracy, enriches contextuality, and boosts the relevance of AI outputs. Compared to other NLP techniques, RAG stands out for its ability to provide more informed and context-aware responses, making it a superior choice for various AI-driven applications.
Other Key Data Concepts to Watch Out For
Beyond RAG, the AI and NLP landscape brims with emerging data concepts like advanced machine learning algorithms, deep learning enhancements, and more. Each of these concepts carries the potential to revolutionize AI further, paving the way for more innovative, more efficient, and more adaptable systems.
Understanding RAG and other data concepts is crucial in grasping the future of AI and NLP. As these technologies continue to evolve, they are set to redefine the way we interact with and leverage AI.
Gain More Insights
As AI continues to evolve, staying informed and engaged is more important than ever. Don’t miss the opportunity to gain more insights from Tiarne. You can connect with Tiarne on LinkedIn here…