Google Teams Up with Welocalize to Test its Adaptive Translation LLM Solution
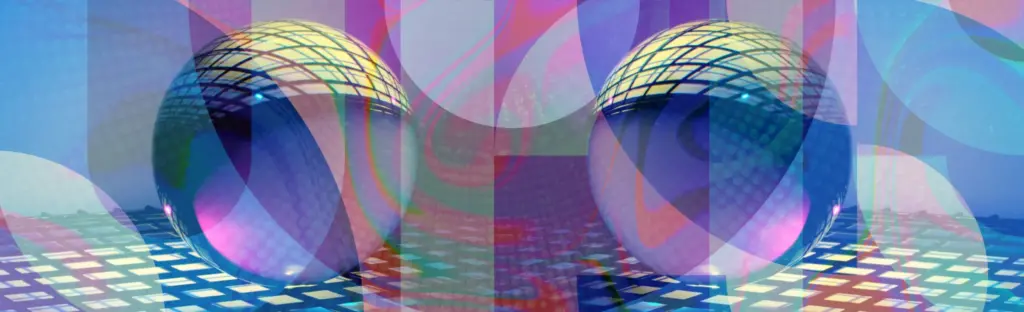
Google has recently collaborated with Welocalize, a prominent language service provider, to rigorously assess its new adaptive translation Large Language Model (LLM) solution. This venture is part of Google’s ongoing commitment to enhance machine translation (MT) through advanced artificial intelligence (AI) technologies.
The partnership’s research highlights the potential for superior LLM outputs, promising enhanced translation accessibility beyond conventional methods. Notably, Google’s adaptive translation system demonstrates superior fluency and tailoring writing styles to meet customer preferences, compared to other evaluated techniques.
Welocalize joined forces with Google in this endeavor, conducting a detailed case study on Google’s adaptive translation LLM solution. This collaboration marks Welocalize as a pioneer in examining these advanced models.
This joint study scrutinizes the early stages of adaptive translation, a novel feature in Google’s Translation API Advanced. This feature integrates a specially fine-tuned LLM for TextTranslation, offering a swift and straightforward means for users to refine translation outputs in real-time, aligning more closely with their stylistic needs and specific use cases. The findings suggest that this approach could lead to higher-quality LLM outputs, enhancing the accessibility of quality translations beyond traditional methods.
Adaptive Translation’s Role in Enhancing Machine Translation
LLMs have transformed machine translation, introducing greater flexibility and contextual understanding. Google leverages this progress by incorporating generative AI models, fine-tuned for specific translation tasks, into their Translation API. These specialized models, derivatives of Google’s foundational models, are designed to tailor translations to particular customer requirements and are accessible in Public Preview via the Translation API Advanced.
Welocalize’s Perspective on Collaborative Research with Google
Mikaela Grace, Head of AI/ML Engineering at Welocalize, shared her insights on the collaboration: “Our joint research with Google reflects our commitment to AI-driven innovation in localization. This venture into adaptive translation with LLMs opens exciting possibilities for enhancing LLM outputs, potentially improving access to high-quality translation beyond traditional workflows.”
Study Goals and Methodology
Welocalize’s study aimed to compare the performance of Google’s adaptive translation LLM solution against its custom and generic MT systems. The research involved selecting existing models with small datasets across various language pairs and content types. It ensured a fair comparison between the Adaptive and AutoML approaches by maintaining equal data volumes. The study included customizing three iterations of Google Adaptive via preview with varying data sizes and involved a detailed human evaluation.
Key Results and Observations
The study revealed that the adaptive translation method outshone traditional methods in terms of accuracy, fluency, style, and adherence to local conventions, with fewer critical errors and enhanced customization to client styles. However, traditional MT models, especially AutoML, excelled in handling terminology and tags. Notably, adaptive translation with smaller example sets scored highest in overall adequacy and fluency, while larger datasets in adaptive translation significantly outperformed AutoML in accuracy, fluency, and style.
Google’s adaptive solution is particularly suited for content types with minimal client-specific terminology, low data for customization, and a focus on style. In contrast, traditional MT models, like AutoML, are more effective for technical writing requiring precise terminology.
In-Depth Analysis of Adaptive Translation Performance
- When fine-tuned with approx. 20,000 segments, the adaptive model strongly outperformed Google’s custom NMT in accuracy, fluency, and style.
- The adaptive model excels at adapting to the customer style from this relatively small dataset, without compromising accuracy.
- When fine-tuned with an even smaller dataset, the Adaptive model strongly outperforms generic Google in accuracy and customer style.
- Client-specific terminology is the main shortcoming of the adaptive model.
The evaluation and comparison of the five models involved assessing adequacy and fluency using a Likert scale of 1-5, where 1 represents poor translation and 5 indicates perfect translation.
Conclusions and Future Directions
This extensive study demonstrates Google’s adaptive translation LLMs’ efficacy in scenarios needing high fluency and style adaptation while identifying areas for improvement in handling client-specific terminology.
As part of Translation API Advanced, adaptive translation facilitates integration with various translation management systems (TMS) and CAT tools, enhancing workflow efficiency. TMS and CAT tool developers are encouraged to update their plugins to include this new feature, allowing customers to benefit from this technological advancement.
Elaine O’Curran, Senior AI Program Manager at Welocalize, commented, “Adaptive models are particularly effective for general, marketing, and creative content. However, until terminology adaptation is refined, we advise against using adaptive translation for projects heavy in specialized terminology, like user guides or help articles with abundant UI references and client-specific terms.”
O’Curran added, “The maximum data size for adaptive models is 30K sentences, which is relatively small for our traditional custom MT models. Our initial hypothesis was that traditional MT struggles with small datasets, and adaptive translation would be a viable alternative. The promising results suggest that adaptive translation might replace traditional MT even with larger datasets. We plan to further explore this with marketing content, for instance.”
Adaptive translation with LLMs represents a potential path to higher-quality LLM outputs, which could result in improved accessibility to quality translation outside of the traditional workflow. Adaptive translation does not require any prompt engineering and will be more easily integrated into existing workflows.
Continuous benchmarking and evaluation of current MT solutions against LLM alternatives is vital for businesses to stay ahead and benefit from emerging GenAI solutions for their global content. This innovation is set to make high-performing and well-tuned MT more widely available.