[On-Demand] 3 Use Cases for GenAI in the Global Patent Process
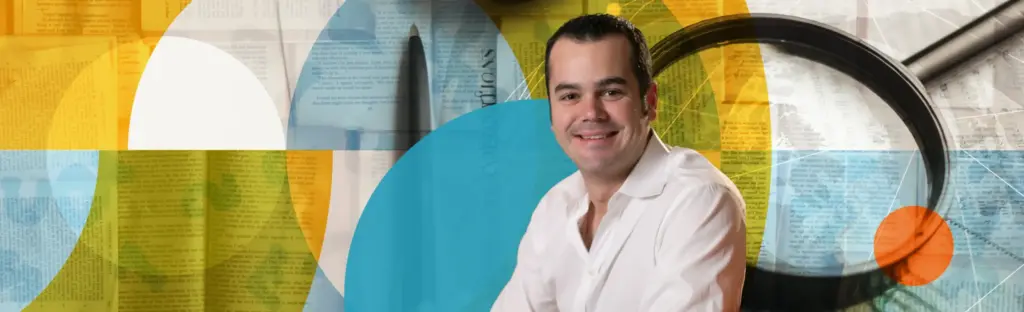
How can patent attorneys and IP professionals leverage the power of generative Al in patents?
In this recorded session, Matt Sekac, Head of AI R&D at Welocalize, delves into the world of Generative Al and its potential impact on the legal and IP industry, specifically, the global patent process.
Sekac sheds light on how Al, specifically large language models (LLMs) like GPT-4, might reshape patent searching, drafting, and translation processes.
3 Use Cases for using GenAI in global patents:
Patent Searching (4.37)
Patent attorneys often sift through existing patents and prior art to ascertain novelty and non-obviousness. Generative Al can synthesize extensive datasets, draw conclusions, and offer insights that could significantly expedite the search process.
Generative AI can hallucinate and bring up incorrect results – so validation is still needed until the technology makes further advancements.
Patent Drafting (6.38)
Although a highly complex and unique process, in the future, LLMs could potentially be used to help attorneys leverage reusable materials, with LLMs synthesizing information from previous patents and contracts.
Sekac addresses some of the risks in this area and cites examples of instances where judges have mandated certificates ensuring human validation of Al-generated content. For drafting and validation, human involvement still remains crucial.
Patent Translation (9.58)
LLMs can be used for translation, and currently, the challenges lie in Al’s fluency and precision. For something as unique as patent applications, where each word is meticulously chosen, accuracy in translation is crucial.
Sekac discusses a hybrid approach with AI tools integrated into a workflow that includes human validation and other types of efficiency tools and technology in order to get the optimal outcome.
About Matt Sekac
Based in New York, Matt Sekac has worked in the global legal and patent space for over 20 years. He currently leads the AI Research and Development Team at Welocalize with a focus on data analytics.
For more information about patent translation and filing coordination, email patents@Welocalize.com
You might also enjoy…
When Can We Trust Generative AI? Podcast with Chris Grebisz, Welocalize CIO
Litkraft vs. GWS: Managing Legal Translation Budgets
Welocalize Guide: The Legal Practitioners Guide to Translation Services
Transcript
Hello, my name is Matt Sekac and I’m the head of AI R&D AI office and data analytics at Welocalize. If you don’t know, Welocalize. Welocalize is one of the largest language service providers in the world. We’re prepare over 2.1 billion words and translate in every year and over 250 languages for some of the largest and most innovative companies in the world. A big part, important part of that process is the work we do in the legal and patent space, where we prepare tens of thousands of patents, translating every year for more than 850 clients ranging from firms and start-up biotech companies all the way up to some of the largest corporate organizations in the world and some of the largest global white shoe firms in the world.
One of the things that we’re always doing at Welocalize is trying to monitor our trends and the spaces where our clients are getting business in terms of technology, and things like that in order to understand how we think our clients are going to be affected and impacted and how we might be able to better support our clients through the evolution of disruptive developments. So today, I want to talk about one such example that has been getting a lot of attention: large language models (LLMs) and generative AI. I want to share some thoughts on how patent practitioners in particular might be able to embrace the disruption that will play out as a consequence of the rapid evolution of this new technology. I’m also going to highlight some of the risks and some of the considerations for what is the best way to actually use this technology and what are ways that this technology might be misused.
Before I joined the innovation organization at Welocalize, I spent more than a decade working as part of our legal and patent services division. I’m not an attorney or a patent practitioner myself. Over the years, I’ve had the opportunity to work with probably hundreds of patent attorneys, agents, paralegals, admins and operations professionals, and that, in turn, is giving me a great deal of opportunity to observe, and I like to think understand how the business of developing patent assets works. You can definitely take everything that I’m about to say with you know, a pinch of salt, but I do think that we are likely to observe an escalating impact on the patent space, specifically from Generative AI technology like GPT-4 (that ChatGPT is currently based on) and then also the parade of similar but I think probably more specialized technology that is likely to follow.
What is Generative AI? Generative AI is a type of machine learning and it’s been trained on a very large amount of data in order to generate outputs based on what it has “learned” from all of that training. Most folks, I think, will be aware of the example of Chat GPT and Chat GPT was trained on something approaching like the entire internet. What Chat GPT is doing is drawing from all of that training data and all of that historical text in order to generate text outputs in response to user prompts.
How can legal professionals use AI in the global patent process? So let me start just by stipulating a couple of things. The first, is I am definitely speculating here. I would like to consider it informed speculation, but the way that things are going, even if everything I say is dead-on, perfect, and on point right now, it’s very possible that in 90 days the whole world will be different. The second thing is: I am not going to be suggesting, at least for the immediate foreseeable future, that the need for human involvement in oversight validation, that that’s going to be wholly negated. It really what I’m talking about is, you know a few areas where I think we’re going to see this increasing impact of generative AI on the global patent process.
So, the first one, the first area where I think we’re going to start to see an impact is search. I think it’s kind of the most obvious application.
When we’re doing a patent search, what a patent attorney is doing is they are engaged in a detailed examination of existing patents and relevant prior art in order to determine the novelty, and non-obviousness of an invention. It’s a pretty clear use case for generative AI, I think, in the sense that large language models and generative AI are doing is to synthesize large quantities of data, summarize them, and even actually draw conclusions. So, I am aware, of course, of some efforts have been made in with some success to apply AI to the patent searching space, the power LLMs bring. It’s almost a reasoning capability in the sense that they allow you not to have too rigidly codify specific queries, specific screens or what sort of logic. You can almost use plain language. And what these tools again are incredibly effective at is synthesizing all of this large data in order to generate relevant outputs. So, taking, for example, a whole corpus of published patent applications and using that to, or evaluating that purpose in the context of, say, a proposed lane 1, in order to identify which applications are most likely to be relevant. Now, one of the risks in something that will keep coming up is what is sometimes referred to as hallucination, and we’ll talk about that more in a second. It might come up with patents that are totally wrong. It will still require validation, but as a sort of first pass or a head start, I think there’s a lot of potential in this technology when it comes to patents search.
The second one is drafting. So I know that this one will probably be met with scepticism and again, I’m not saying, at least in the immediately foreseeable future, that I think there’s going to be no need at all for human involvement in patent drafting or really the generation of any other or high stakes or regulated content. But when you think about what you’re trying to do when drafting a patent, you’re trying to craft a well supported set of claims that secures maximum protection without running afoul of prior art or patentability regulations, again requiring the synthesis in the application of a massive amount of information. So legal instruments like contracts, for example, are probably more obvious. They contain, I think, more boilerplate material. Again, there’s already a lot of instrumentation and technology out there to facilitate the, the reuse of elements of contracts and the repurposing of previously utilized material in patents, of course, are by definition novel they’re, by definition unique, and it needs to be crafted with very special care in the context of, again, the prior art and relevant regulations. But in the end, I do think large language models will still prove helpful as long as they’re used the right way. So, there are again, you know, talking about the RISKS.
There have been a couple of pretty high-profile, well-covered examples of large language models hallucinating, so to speak, or sort of saying the wrong thing. The one really widely covered example was in the southern district of New York where you had an attorney submit a brief to the court that was written by Chat GPT and what it had done was come up with a bunch of case citations that were not actually real cases and I believe in the end the attorney there, ended up being sanctioned. But to my mind, that’s not an example, or that doesn’t make the case that this technology can’t be used. It’s an example of how it’s used the wrong way when you look at the another widely publicized example, which is the judge in Texas who had a requirement that all attorneys appearing before the court have to file a certificate now, which tests you know basically that Chat GPT or some other tool like that wasn’t used to prepare the court filing. If you actually read what the certificate says, it says either that no portion of the filing was drafted by generative AI, or that that any language drafted by generative AI was checked for accuracy by a human being.
So even in that example, there is a recognition that it can be used the right way in order to make, in order to make professionals in general more efficient.
So the third area is translation. We’re a language services provider, a translation company, and when we think about translation, and translation for patents in particular, I think in some ways the discussion is pretty akin to drafting, in the sense that the real test is going to end up being how best to deploy, how best to integrate this technology in order to deliver the required outcomes most efficiently.
One of the things about large language models and generative AI is the way that they actually work. What they’re actually trying to do is come up with the next word. They’re always just thinking about the next word. So, what that means is, if you ask a tool like Chat GPT to translate of segments of text or a paragraph into French, it’s not exactly trying to translate it into French. What it’s actually doing is it’s trying to write that text again in French. That’s kind of the same thing, but it’s not exactly the same thing. That’s where it might decide to choose different words or different sentence structure or things like that, because again, it’s job is to take what you give it as an input and give that output back, but this time in French or whatever other language. For some applications, that might actually be better. What we find is that tools like GPT 4 and Chat GPT do really well with what we call fluency. They sound natural, but they sometimes struggle with accuracy. Again, they make mistakes.
When we think about using technology like this on a patent, that might be worrisome, right, because in patents…very, very meticulously, very, very carefully, every word is chosen specifically, in the order of those words chosen specifically, but there might still be waves and I believe there will be ways to integrate this technology into a workflow that includes human validation and other types of efficiency tools and technology in order to get to the right outcome and sort of, and so our ability to identify potential issues or just almost as a check, that kind of helped validate that the output is what we need to prepare an application for filing in a place like japan or Korea or Mexico or Europe or wherever.
In the end, I think that the power and the potential of large language models and generative AI, it’s, I think it’s going to be too great for anyone in any professional space, to expect that it’ll just sit and stay locked away forever.
It’s going to keep evolving, it’s going to keep getting better. I think that the test of all of language and localization service providers, patent practitioners, and almost everyone else it’s going to be: how effectively do we put this technology to use, how do we use it to make us better? And at Welocalize. That’s what we’re thinking about. Our job is ultimately to help clients achieve their goals. And all of this frenzy around generative AI, I think it is a justified frenzy, but it really boils down to: Okay, so what? What do we do with it? How do we incorporate it into the work we’re doing? How does it make us better? How does it help us help our clients be better?
At Welocalize, we are laser-focused, moving quickly, and leading the industry in the development and application of this technology, and we would love to hear ideas and feedback from you on what outcomes we can help you achieve. So, thank you for watching and for listening. If you want to reach out and find out more about our work in this area our email, will be here on the screen, and let’s keep the conversation going. Thank you again.